How can AI forget?
Machine unlearning is an emerging field in AI that addresses the challenge of forgetting information in machine learning models. With lawsuits and privacy concerns on the rise, the ability for ML systems to efficiently remove data has become crucial for businesses. The future of machine unlearning involves advancements in hardware, policy, and regulation, and increased public awareness.
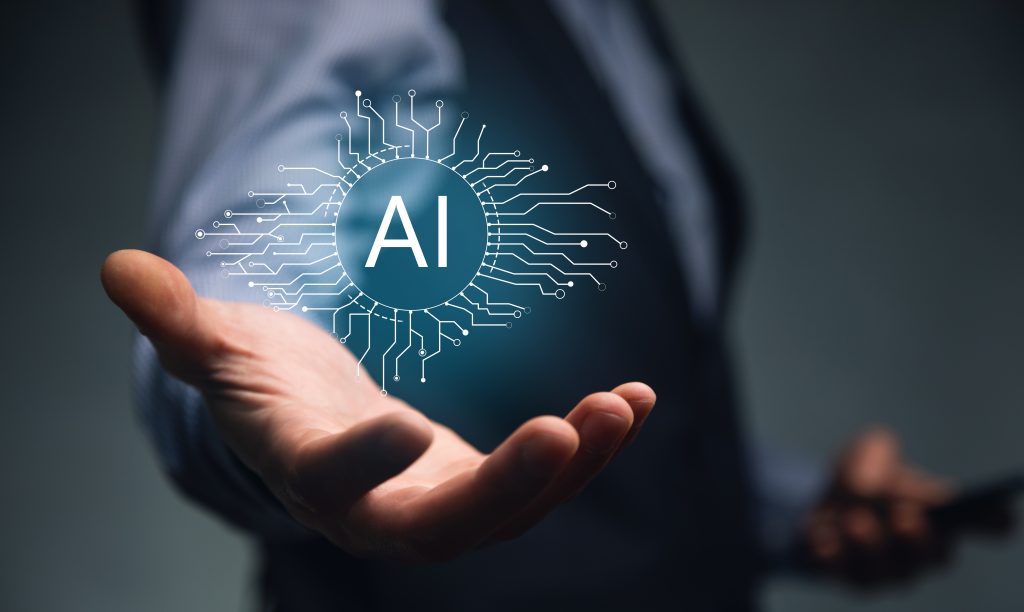
AI does not forget. This presents a problem when AI platforms are trained on outdated, incorrect, or private data.
With the growing number of lawsuits filed against companies over the use of certain data in machine learning (ML) systems, the need for ML models to efficiently forget or erase information has become significant for privacy, security, and ethics.
AI-unlearning is the process of erasing the influence of specific datasets on an ML system. When concerns arise with a dataset, it is often modified or deleted. However, when the data has been used to train a model, it becomes challenging to understand how specific datasets have impacted the model during training and even harder to undo the effects of a problematic dataset. ML models are essentially black boxes, making it difficult to decipher the exact impact of individual datasets.
OpenAI, the creators of ChatGPT, and generative AI art tools, have faced criticism and legal battles concerning their training data. Privacy concerns have also been raised after membership inference attacks revealed that it is possible to infer whether specific data was used to train a model, potentially compromising individuals’ privacy.
While machine unlearning may not prevent companies from legal disputes, it can help bolster their defence by demonstrating that datasets of concern have been entirely removed. A straightforward solution for producing an unlearned model is to identify problematic datasets, exclude them, and retrain the entire model from scratch.
However, this brute-force approach is costly and time-consuming. The main objective of machine unlearning is to forget undesirable data while retaining the model’s utility, all done with high efficiency. It is pointless to develop a machine unlearning algorithm that consumes more energy than retraining the model.
Machine unlearning faces several challenges, including efficiency, standardization, efficacy, privacy, compatibility, and scalability. These challenges need to be addressed to achieve a balance between efficient unlearning and the overall progression of the field. Employing interdisciplinary teams consisting of AI experts, data privacy lawyers, and ethicists can help identify potential risks and monitor progress in the machine-learning field.
The future of machine unlearning involves Google’s machine unlearning challenge, which aims to unify and standardise evaluation metrics for unlearning algorithms and foster innovative solutions to the problem. Additionally, the increasing number of lawsuits against AI and ML companies is expected to drive further action within these organizations.
Looking ahead, advancements in hardware and infrastructure are anticipated to support the computational requirements of machine unlearning. Increased interdisciplinary collaboration between AI researchers, legal professionals, ethicists, and data privacy experts may streamline the development and implementation of unlearning algorithms. Lawmakers and regulators may also focus on machine unlearning, potentially leading to new policies and regulations. Increased public awareness of data privacy issues may also influence the development and application of machine unlearning.
For businesses implementing or using AI models trained on large datasets, it is crucial to understand the value of machine unlearning. Monitoring recent research, implementing data handling rules, considering interdisciplinary teams, and considering retraining costs are actionable insights to manage data-related issues. Keeping pace with machine unlearning is a proactive long-term strategy for businesses using large datasets to train AI models.
In conclusion, machine unlearning plays a vital role in responsible AI by improving data handling capabilities while maintaining the quality of ML models. Adopting and implementing machine unlearning is becoming a necessity for businesses in the evolving AI landscape.
With the philosophy of responsible AI emphasising transparency, accountability, and user privacy, machine unlearning aligns with these principles. Although the field is still developing, as evaluation metrics become standardised, implementing machine unlearning will become more manageable. Businesses should proactively address the challenges associated with data handling and strive to incorporate machine unlearning practices in their AI models and large datasets.