AI in science: Potential and risks
AI has the potential to revolutionise science by producing readable research summaries, analysing vast amounts of data, and suggesting new drugs and materials. However, there are downsides, including academic misconduct, deceptive data, and biases in AI models. Instances of misconduct, such as not acknowledging the use of language models, have increased. The quality of research can be compromised when data is polluted by AI, and it is difficult to identify machine-generated content. AI models can face limitations and concerns arise when computer-generated insights come from opaque models. The incentives and threats posed by AI in science mirror those of human researchers.
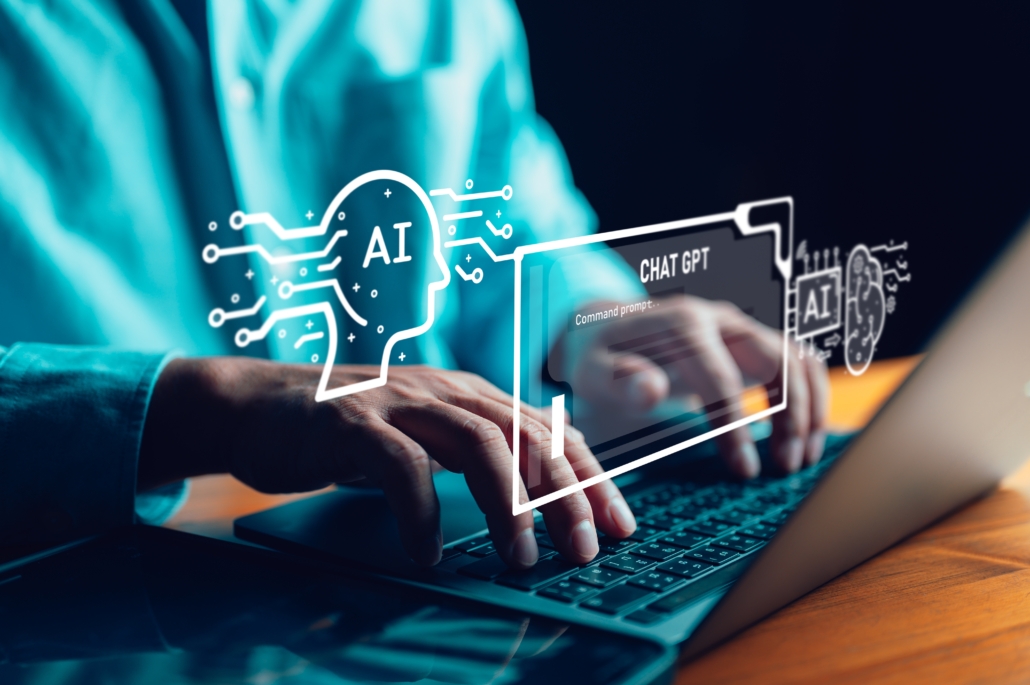
AI has the potential to revolutionise the field of science by aiding in research and generating new insights. However, there are also downsides and challenges to consider when integrating AI into scientific practices. One significant problem is academic misconduct, where AI tools are misused or not properly credited. Some researchers have used language models (LLMs) to write research papers without acknowledging their contributions, potentially deceiving others. The use of LLMs in research papers has increased, and phrases like ‘regenerate response’ suggest the undisclosed use of LLMs.
The integrity of research data is also a concern. AI can taint the quality of data when used in research. For example, researchers who employed remote workers through platforms like Mechanical Turk found that over a third of the responses they received were produced with the assistance of chatbots. This compromises the reliability of research, particularly in disciplines like social sciences that heavily rely on platforms like Mechanical Turk.
Manipulating text is not the only issue; AI can also manipulate images. Identical image features have been found in scientific papers, suggesting the use of AI-generated images to support research conclusions. Currently, there is no reliable method to distinguish machine-generated content from human-generated content, whether it be text or images.
Training AI models present limitations and challenges as well. Using somewhat old data to train models can hinder keeping up with the fast-paced advancements in scientific fields. Training models on their own outputs can lead to ‘model collapse,’ where the quality and variety of the produced results significantly deteriorate.
The lack of transparency in AI models is another concern. Machine-learning systems are considered ‘black boxes’ as their inner workings are often difficult for humans to understand. This lack of transparency raises questions about the reliability of the insights generated by these models. However, researchers argue that despite being unexplainable, these models can still be useful if their outputs undergo rigorous testing and verification in real-world scenarios.
Source: The Economist