Debating Technology / Davos 2025
23 Jan 2025 08:00h - 08:45h
Debating Technology / Davos 2025
Session at a Glance
Summary
This discussion focused on the current state and future of artificial intelligence (AI), exploring its potential benefits, challenges, and ethical considerations. The participants, Dava Newman from MIT and Yann LeCun from Meta, debated various aspects of AI development and implementation.
LeCun argued that current large language models (LLMs) are limited in their capabilities and will likely be replaced by more advanced systems within 3-5 years. He emphasized the need for AI to understand the physical world, not just manipulate language. Newman stressed the importance of human-centered design and ensuring AI systems are trusted, responsible, and representative of diverse populations.
The debate touched on the challenges of open-sourcing AI technology, balancing innovation with safety concerns. Both speakers agreed on the need for transparency and accountability in AI development. The discussion also explored the role of values in AI systems and the difficulties in implementing universal values across diverse cultures.
Newman highlighted the potential of AI in space exploration and personalized medicine, while LeCun focused on the future of robotics and the need for more efficient AI systems. The speakers disagreed on the near-term potential of brain-computer interfaces, with Newman arguing that such technology is already in use for prosthetics.
The discussion concluded with reflections on the importance of involving the next generation in AI development and the potential for AI to revolutionize various fields, including healthcare and sustainable technology. Both speakers emphasized the need for responsible development and implementation of AI technologies to ensure they benefit humanity and the planet.
Keypoints
Major discussion points:
– The current state and future potential of AI technologies like large language models
– Balancing open source development with safety and ethical concerns
– The need for AI systems to have values and be human-centered
– Challenges of developing AI for a diverse global population with different cultural values
– Potential applications and impacts of AI in areas like space exploration, robotics, and healthcare
The overall purpose of the discussion was to explore both the exciting potential and concerning challenges posed by rapidly advancing AI technologies, and to consider how to develop these technologies responsibly in a way that benefits humanity.
The tone of the discussion was largely thoughtful and measured, with the speakers acknowledging both the promise and risks of AI. There was a sense of cautious optimism, tempered by calls for responsible development and human-centered design. The tone became somewhat more enthusiastic and forward-looking towards the end when discussing future applications and possibilities.
Speakers
– Moderator: No specific expertise or role mentioned
– Dava Newman: Head of the MIT Media Lab
– Yann LeCun: Leads AI research and other activities at Meta
Additional speakers:
– Mukesh: From Bangalore, India (audience member)
– Moritz Berlenz: From Lightspeed (audience member)
– Martina Hirayama: State Secretary for Education, Research, and Innovation, Switzerland (audience member)
– Mukta Joshi: From London (audience member)
Full session report
Revised Summary of AI Discussion Town Hall
This summary covers a town hall discussion on artificial intelligence (AI) at a conference, featuring Dava Newman, head of the MIT Media Lab, and Yann LeCun, who leads AI research at Meta. The conversation, moderated and including audience participation via live stream and Slido, explored the current state, future potential, and ethical considerations of AI development.
Current State and Future of AI Technology
A key debate centered on the present capabilities and future trajectory of AI, particularly large language models (LLMs). LeCun argued that current LLMs have significant limitations and will likely be replaced by more advanced systems within 3-5 years. He emphasized the need for AI to progress beyond text manipulation to understand the physical world and sensory data. LeCun also noted the intrinsic unsafety of LLMs due to their uncontrollability. Newman, while acknowledging AI’s current limitations, framed the technology as still in its infancy, especially compared to human capabilities.
Open Source Development and Diversity in AI
Both speakers stressed the importance of open source development and diversity in creating beneficial AI systems. LeCun emphasized that open source and diversity are crucial to developing AI that can represent global values. He argued that open source development is essential for transparency and for allowing a wide range of contributors to shape AI technologies. Newman echoed this sentiment, highlighting the need for diverse perspectives in AI development.
Values and Ethics in AI Development
A significant portion of the discussion focused on implementing values and ethics in AI systems. Newman emphasized the importance of human-centered design and clearly articulated values in AI development. She stressed the need for transparency and trust in the process. LeCun acknowledged the challenge of implementing diverse global values in AI systems, given varying cultural contexts worldwide. Both speakers agreed on the necessity of incorporating ethical considerations into AI development from the outset.
Content Moderation and AI
The conversation touched on the evolution of content moderation approaches, particularly at Meta. LeCun highlighted improvements in AI-driven moderation and explained current policies. Newman argued for the need for better policies to address harmful content, illustrating the complexity of balancing free speech with content moderation.
Emerging AI Applications and Societal Impact
The discussion explored various emerging applications of AI and their potential societal impact. Newman highlighted the potential of brain-computer interfaces, particularly in prosthetics, and AI’s role in space exploration and the search for extraterrestrial life. She also emphasized AI’s potential in personalized medicine and healthcare. LeCun focused on the importance of efficiency and power consumption in AI development, especially in robotics and large-scale AI systems.
Challenges and Concerns
Several challenges and concerns were raised throughout the discussion, including:
1. Balancing open source development with potential misuse of AI technologies
2. Ensuring safety and alignment of increasingly autonomous AI systems
3. Addressing concerns over AI’s impact on employment and society
4. Implementing diverse global values in AI systems effectively
5. Determining appropriate thresholds for content moderation on social platforms
Audience Engagement
The moderator incorporated audience questions and presented a word cloud generated from audience responses about AI trust and safety. This interactive element provided insight into public perceptions and concerns about AI development.
Conclusion
The town hall discussion offered a comprehensive overview of the current state and future challenges of AI development. It highlighted the need for responsible innovation that respects diverse values and cultures while pushing the boundaries of technological capabilities. The interplay between technical limitations, future research directions, and ethical considerations provided a nuanced view of the complex landscape of AI development and its potential impact on society.
As AI continues to advance, ongoing dialogue and collaboration will be crucial to address the challenges and opportunities presented by this transformative technology. The discussion underscored the importance of involving diverse perspectives, including the next generation, in shaping the future of AI to ensure it benefits humanity and the planet.
Session Transcript
Moderator: There’s so much to talk about in technology. Now, the title is debating technology. I don’t think there’s a debate technology, yes or no. Um, but in covering Silicon Valley for 25 years, I often hear, you know, technology can be used for good or bad, which is inherently true. But sometimes that’s used to say, especially by the makers of the technology, well, it’s gonna be used for good or bad. Hopefully the good outweighs the bad. And to me, that neglects our responsibility to push and steer and limit the technology so it is used for good. Um, but we’re gonna talk about it. This is a moment of great excitement, especially with artificial intelligence, robotics, and all these technologies. Um, but it’s also a moment of great concern. A lot of people have legitimate fears about what this change will bring. Uh, that’s enough from me. I’m excited to be joined by Dava Newman, head of the MIT Media Lab, and Yann LeCun, who leads AI, uh, research and other activities at Meta. Um, David, maybe to start with you, I mean, you have such a broad background in technology from obviously your experience in space. Where is your head these days? What are the problems, uh, and areas that you think need our attention? And where are you wrestling your brain around?
Dava Newman: Thank you, everyone. Good morning. Pleasure to, to be with you. So where’s my brain? Typically in outer space, you know, thinking about becoming an, uh, you know, uh, interspecies, uh, will we find life elsewhere? It’s not option B. So where my head really is in thinking about technology and the disruption that we feel and that much more orders of magnitude more disruption that’s coming. So maybe I’m paint the picture. It really is, I think, uh, uh, you know, technology super cycle now convergence of probably three technologies at once. You know, the industrial revolution, that was, it was okay when we put one technology at a time. Gen AI took me 30 seconds before getting into this. It’s, it’s coming. Large languages. It’s, it’s But it still is an infancy. At the MIT Media Lab, we’ve been working on AI for 50 years. So now that it’s common in everyone’s hands, a co-pilot, I’m sure we’re going to debate that and talk a lot about it with my esteemed colleague and expert developing that. We’re doing a lot. Most important thing I want to emphasize just in the introductory about AI and Gen AIs, we design for humans, human-centered, human-flourishing at the Media Lab. So is it trusted? Is it responsible? That’s the premise. Actually, we don’t do it if it’s not. But hold on to your seats, everyone. Rocket launch is coming soon. Soon, I think we’ll all be talking about GenBio. If you’re not already, not just synthetic bio, but generative bio. We don’t, biology is organic. So when AI morphs into GenBio, it’s no longer a large language model. What we’re working on actually, Media Lab, large nature models. Now you’re ingesting biology and genetics and biological. Wrap that all around into sensors. Internet of things. We’re pretty famous for, I call it now, internet of all things. Because of IoT for the oceans, to monitor all biodiversity, for the land, climate, air, atmosphere, you might think of, and from space. More than half of all of our climate variables are now measured from space. So hopefully, that kind of technological whirlwind, I don’t know what else to call it, is coming with GenAI, GenBio sensors, measuring everything. To finish up, I put humans and human-centered design right in the middle. And asking the upfront questions. Is it intentional for human flourishing and all living things flourishing? If the answer to that is no with our algorithms, then I don’t think we should be doing it.
Moderator: And Jan, that’s a good point to turn to you. How do we make sure the AI we can build is the AI we want? How are you trying to focus your work and the development at Meta to make sure that? that we get an AI that works for humanity?
Yann LeCun: There’s two answers to this. The first thing is you try to make it work well and reliably, and the flavor of generative AI or AI that we have at the moment is not quite where we want it to be in terms of it’s very useful, we should push it, we’re pushing it, trying to make it more reliable, trying to make it applicable to a wide range of areas, but it’s not where we want it to be and it’s not very controllable for various reasons. So I think what’s gonna happen is that within the next three to five years, we’re gonna see the emergence of a new brand or paradigm for AI architectures, if you want, which may not have the limitations of current AI systems. So what are limitations of current AI systems? There are four things that are essential to intelligent behavior that they really don’t do very well. One is understanding the physical world. Second one is having persistent memory. And third and fourth are being capable of reasoning and complex planning. And LLMs really are not capable of any of this. There is a little bit of an attempt to kind of bolt some warts on them to kind of get them to do a little bit of this, but ultimately this will have to be done in a different manner. So there’s gonna be another revolution of AI over the next few years. And we may have to change the name of it because it’s probably not going to be generative in the sense that we understand it today. So that’s a first point. Some people have called this in different names. So the technology we have today, large language models, deals very well with the discrete world and language is discrete. I don’t want to upset Stephen, who is a Syrian thinker, who is in the room here, but. To some extent, language is simple, much simpler than understanding the real world, which is why we have AI systems that can pass the bar exam or solve equations and things like that, do pretty amazing things. But we don’t have robots that can do what a cat can do. The understanding of the physical world of a cat is way superior to everything we can do with AI. So that tells you the physical world is just way more complicated than human language. And it’s because, why is language simple? It’s because it’s discrete objects, and the same with DNA and proteins, right, it’s discrete. So the application of those generative methods to this kind of data has been incredibly successful because it’s easy to make predictions in a discrete world. You can never predict what word will come after a particular text, but you can produce a probability distribution of all possible words in the dictionary, and there’s only a finite number of them. If you want to apply the same principle to understanding the physical world, you will have to train a system to predict videos, for example, right, show a video to the system and ask it to predict what’s going to happen next. And that turns out to be a completely intractable task. So the techniques that are used for large language models do not apply to video prediction. So we have to use new techniques, which is what we’re working on at Meta, but it may take a few years before that pans out. So that’s kind of the first thing. And when that pans out, it will open the door to a brand new class of applications of AI because we’ll have systems that will be able to reason and plan, because they will have some mental model of the world that current systems really don’t have. So they’ll be able to predict the consequences of their actions and then plan a sequence of actions to arrive at a particular objective. And that may open the door to real agentic system, where you’re talking about agentic AI. knows how to do it. And that’s kind of one way to do it properly. And also to robotics. So the coming decade may be the decade of robotics. Because that was the first answer. And the second answer, which is shorter, the way to make sure that AI is applied properly is to give the tools for people to build a diverse set of AI systems and assistants which understand all the languages in the world, all the cultures, value systems, et cetera. And that can only be done through open source platforms. So I’m a big believer in the idea that the way the AI industry and ecosystem is going, open source foundation models are going to be dominant over proprietary systems. And they’re going to basically be the substrate for the entire industry. They already are, to some extent. And they are going to enable a really wide diversity of AI systems. And I think it’s crucially important, because within a few years, you and I both are wearing those smart glasses, right? And you can talk to an AI assistant using those things and ask any question. But pretty soon, we’re going to have more and more of those things with displays in them and everything. And all of our digital diet will be mediated by AI assistants. And so if we only have access to three or four of those assistants coming from a couple of companies on the west coast of the US or China, it’s not going to be good for cultural diversity, democracy, everything else. We need a very wide diversity of AI assistants. That can only happen with open source, which is what Meta has been promoting as well.
Moderator: Well, thank you both. I think that sets us up well for a discussion. And as a reminder, this is a town hall, not a panel. So we’re going to be bringing in both the audience here in this room of incredible guests, as well as those on the live stream. So the first thing we did is we asked the folks on the live stream. There is a Slido you can join. Also, we asked, how would you like these emerging technologies to contribute to the future? And we’re not going to show all the answers, but here’s a word cloud of some of what folks have said. So if we just quickly look at that. Well, that’s the question. I’m not quite sure how we get to the answer. All right, well, I’m sure people talked a lot about both what they’re excited about and what they’re worried about. I want you to get ready with your questions in the room. I’m sure everyone has some. But Yann, I want to follow up on the open source thing, because there’s really a big debate. I mean, as I said, technology is not a debate, but the approaches we take. And certainly, open source has all the advantages that you mentioned. It allows people all over the world to join in. Only a few people are going to be able to train one of these giant models, but a lot of people can make use of them and can contribute. At the same time, there’s a real concern that taking this powerful technology and giving it to the world and saying, basically, here’s our acceptable use policy. Here’s what you can and can’t do. But to be honest, there’s really no way of enforcing that. Once it’s out, it’s out. How do we make sure something is both open source and safe?
Yann LeCun: So what we do at Meta is that when we distribute a model, so by the way, we say open source, but we know technically those things are not really open source, because the source code is available. The weights of the model are available for free and you can use them for whatever you want, except for those restriction clauses. Don’t use it for dangerous things. So, the way we do this is that we fine-tune those systems and red-team them to make sure that at least to first order, they’re not, you know, kind of spewing complete nonsense and or toxic answers or things like that. But there is a limit to how well that works, and those systems can be jailbroken. You can do what’s called prompt injections or type of prompt that will basically take the system outside of the domain where it’s been fine-tuned, and, you know, you’re going to get to its, you know, kind of root things, and then that depends on what training data is being pre-trained on, which, of course, is a combination of high-quality data and not-so-high-quality data.
Moderator: And Dava is putting something like that into the world. I mean, obviously, there are benefits to open sourcing that way. MIT is a pioneer in open source. There’s an MIT license for open source. I can’t remember. It may even be the license that Meta uses. At the same time, when you talk about having this technology be human-centered and putting humans and our needs and concerns at the forefront, what do you think needs to be done? You talked about synthetic biology and, you know, all these things. Obviously, there’s a lot, you know, there’s a lot of neglected diseases. There’s a lot of things we want to use these new technologies for, and we don’t want everyone just in their home developing new microorganisms to run around. So what are your thoughts on how we make this technology broadly available but still safe?
Dava Newman: Yeah, thanks. That’s the question, and seeing what people are concerned about, too, AI in space. I agree with that. We can talk about the word cloud. But so, you know, based on open source platforms but with guardrails, and we have to be all held accountable, right now we can, you know, ask the audience as well, you know, does AI work for you? And what I mean, do you trust it? Is it responsible? Is it representative? Do you think it has the training data that represents you?
Moderator: Well, let’s ask the audience. How many of you feel that…
Dava Newman: Do you think it’s safe, secure, and, you know, you’re going to launch in and use it today, you know, during this debate? Anyone raise their hand?
Moderator: Well, I think there’s the answer, and I think it’s not… How many people would be open to AI, would love to use AI once they do feel it’s safe and secure?
Dava Newman: So, that’s why I asked the question. So, it’s not there yet. So, it’s not representative, doesn’t represent everyone in this room. The world is much more diverse than what we have in the room, so it doesn’t work. So, maybe this is where the debate starts. So, we’re, you know, open source. We want to be open source. We want all the… You know, all my students are superstars and geniuses. We want all the next generation of the world to be able to give their creativity, their curiosity, because that’s how human flourishing happens. But if we just let the algorithms, again, on their own, I think that we really have to rethink, where does the training data come from? Where is the transparency? Where is the transparency? Does it work for all of us? I think if those questions are answered, oh, we have the majority of the folks, you know, opting in and hopefully making it better, right? Open sourcing it is because you can get all the good ideas and enhancing. So, we see that, you know, coming, enhancing it, making it work for everyone, but I think we, you know, here are very intentional. Where is the transparency? Where is the trust? You know, has it kind of gotten away from us? So, these are really important questions.
Moderator: And, Yann, I want to push you one more time, and then I really, I hope you all have your questions ready, because I’m coming to you next. I want to push you one more area, which is values, and I wrote about this last year that, you know, social media has been about content moderation. What speech do you allow? Where do you draw the lines? Obviously, you know, it’s something that Meta has spent a lot of time on, has had different approaches, but it strikes me that these AI systems are going to have to have values, and I wrote that, you know, your PC doesn’t really have a set of values. values. Your smartphone, you know, yes, there’s some app store moderation, so, you know, at the extreme, there’s some limits. But the AI system is going to answer the hard questions. And, you know, how do we do that in a world where, you know, people in the Middle East have different values than people in the U.S.? People in the U.S. have different values than people in the U.S. Recently, Meta made a bunch of changes to how it’s going to approach that, allowing a lot more speech even that might be considered very offensive, distasteful, even dehumanizing. Where is the role of the tech companies in putting their thumb on the scale of the values? You know, how much pressure is there going to be from governments to control what speech, how AI chatbots, for example, answer questions around gender, sexuality, human rights?
Yann LeCun: So there is an interesting debate about this. So this is not my specialty, I should tell you, but it’s an interesting topic nevertheless that I’m interested in. So Meta has gone through several phases concerning content moderation and how best to do it. And including with questions not just about toxic content, but also about disinformation, which is a much more difficult problem to deal with. So until 2017, let’s say, detecting things like hate speech on social networks was very difficult because the technology just wasn’t up to snuff. And counting on users to flag objectionable content and then have it reviewed by humans just doesn’t scale, particularly if you need those humans to speak every language in the world. And so that just wasn’t technologically possible. You just couldn’t do it. And then what’s happened is that there’s been this enormous progress in natural language understanding. since 2017, basically, and that has made an enormous amount of progress. So now detecting hate speech in every language in the world is basically possible with some good level of reliability. So the proportion of hate speech, for example, that is taken down automatically by AI system was on the order of 20 to 25% late 2017, late 2022, five years later, because of transformers, all the stuff that everybody is excited about today, it was 96%. Now that probably went too far, because the number of false positives, of good content that was taken down, is probably pretty high. So there are countries where people just want to kill each other, and you probably want to kind of calm down, so put the detection threshold pretty low. Countries where there is an election, and things kind of rile up, so also you want to lower the threshold of detection, so that more things get taken down, to sort of calm people down. But then most of the time, you want people to be able to debate important societal question, including for questions that are very controversial, like gender and political opinions, somewhat extremes. And so what’s happened recently is the company realized it went a little too far, and there were just too many false positives. And now the detection thresholds are going to be changed a little bit to authorize discussions about topics that are big questions of society, even if the topic is offensive to some people. So that’s a big change, but it doesn’t mean content moderation is going to go away, it’s just there, it’s just you change the threshold. And again, the answer is different in different countries. So in Europe, it’s illegal, hate speech is illegal, neo-Nazi propaganda is illegal. You have to do it for legal reason, you have to moderate that for legal reason, not so in the US. In various countries, you have different standards. as you said. Then there is a question of disinformation. And there, until now, Meta used a fact-checking organization to fact-check the big posts that had gathered a lot of attention. But it turns out this system doesn’t work very well. It doesn’t scale. You don’t have a large coverage of the content that is being posted because those organizations, there’s only a few of them and they have a few people working for them. And so they can’t just debunk every, you know, dangerous misinformation that circulates on social networks. So the system that is being implemented now that will be rolled out is crowd sourcing. Essentially have people themselves, you know, kind of write comments on posts that are controversial. And that is likely to have much better coverage. There are some studies that show that this is a better way of doing content moderation, particularly if you have some sort of karma system where people who make comments that turn out to be reliable or liked by other people, so they get promoted. Several forums have used this system for many years. So the hope with Meta is that this will actually work better. And it also has a big advantage, which is that Meta has never seen itself as having the legitimacy to decide what is right or wrong for society. And so in the past has asked governments to regulate. Asked governments around the world. This was during the first Trump administration. Tell us what is acceptable on social networks for online discussion. And the answer was crickets. There was basically no answer. I think there was some discussion with the Macron government in France, but the Trump administration at the time, the first one said, we’re the first amendment here. Go away, you’re on your own. So all those policies kind of resulted from this absence of regulatory environment, and now it’s crowdsourced, it’s, you know, content moderation for the people by the people.
Moderator: Well there’s much more we could talk about, but I don’t want to… Yes. Oh, yes, please, Dava.
Dava Newman: If I could get us back to values, I think that’s the right question. So if we can, that should be the first question, what are the values? So, and you have to be able to articulate your values, articulate my values, it’s up to leadership to articulate values, so, you know, for me, I mean, it’s integrity, excellence, curiosity, community, community encompasses belonging, and collaboration. So if you can articulate your values, and then as designers, as builders, as technologists, flow from those values, we can get it right. What if we get this right? So I think you really, we need to back up. So I mean, I should articulate, and in the, you know, there’s checking, what are the values? Do we have aligned values? Then we can collaborate, then we can all collaborate and work together and respect our cultural differences and all the, you know, the cornucopia that humanity is, and that’s wonderful, and that’s the opportunities to go across, you know, all the cultures, but I think we fundamentally still have to have the discussion about values, and do we share values? That’s the, I think, fundamental first question.
Yann LeCun: Yeah, the core shared values that, you know, need to be expressed, I mean, in that sense, the content policy for META are published, right, so it’s not a secret, but then there is the implementation of it, right, and META in the past has made a mistake, deployed the system, and then realized that this is not working the way we wanted it, so kind of rolled it back and replaced it by other systems. It’s constantly-
Dava Newman: But you could lead, you could lead an industry, and, you know, lead in, and be out in the front without discussion.
Yann LeCun: By all measures, actually, META is leading in terms of content moderation, absolutely.
Moderator: And Dava, is that your sense? I mean, are you concerned with- the new policies that, you know, I mean, obviously, it’s very difficult to say what our shared values are. A lot of debates, again, even in the U.S., at the same time, you know, we talked about a human-centered world, and the new policies certainly allow a lot of dehumanizing speech, whether it’s comparing women to objects, trans people to it, gay people mentally ill. Have they gotten that balance right, or are they going in the wrong direction?
Dava Newman: We don’t have the right policies, absolutely no, emphatically no. We know what’s wrong and right. We know human behavior. We know civility. We know what makes you happy when you’re teaching your kids, and we should probably look at our children, our kids, and the young generation as well, especially when we talk about values and what we have and, you know, who we aspire to be. There’s a chance to get it right, but, you know, we’ve run the experiment, you know, Internet One, Internet Two. I think we’re running the experiment, so this is the opportunity to get it right.
Moderator: I want to bring in the audience. Who would like to build on the discussion we’ve had? And please just say your name and where you’re from. There’s a mic coming around, but keep the intro short and ask a question.
Audience: I’m Mukesh from Bangalore, India. So Jan, your group is at the forefront of AI research, and so are many other groups around the world. Do we know where we are going? Is there a mental model of five years from now, because we’re all speculating and asking questions about where AI is today, challenges and so on. Do we understand where we’re going enough to have some prediction about five years, or is it just too much wide open?
Yann LeCun: So my colleagues and I certainly understand where we are going. I can’t claim to understand what other people are doing, particularly the ones that are not publishing their research and basically, you know, have crammed up in recent times. But the way I see things going, so first of all, I think the shelf life. of the current paradigm, large language model, is fairly short, probably three to five years. I think within five years, nobody in their right mind will use them anymore, at least not as kind of the central component of an AI system. One analogy that some people have made, which I’ve recycled, is LLMs are good at manipulating language, but not at thinking, okay? Manipulating language is done by a little piece of the brain right here called the Borca area. It’s about this big and only popped up in the last few hundred thousand years. It can be that complicated. What about this? The frontal cortex, that’s where we think, right? We don’t know how to reproduce this. So that’s what we’re working on, having systems sort of build mental models of the world. So if the plan that we’re working on succeeds with the timetable that we hope, within three to five years, we’ll have systems that are a completely different paradigm. They may have some level of common sense. They may be able to learn how the world works from observing the world go by and maybe interacting with it. You know, deal with real world, not just a discrete world, and open the door to another application. I want to give you just a very interesting calculation. A typical foundational model today, large language model, is trained on 20 trillion tokens or 30 trillion tokens. A token is typically three bytes. So that’s about, you know, 9 times 10 to the 13 bytes, 10 to the 14 bytes, okay, let’s round it up. This basically is almost all of publicly available text on the Internet. It would take any of us several hundred thousand years to read through it, okay? Now compare this with what a four-year-old has seen in the four years of life. You can put a number on how much information gets to the visual cortex or through touch, if you’re blind. And it’s about two megabytes per second, about one megabyte per optic nerve, about one byte per second per optic nerve. optic nerve fiber. We have one million of them for each eye. Multiply this by four years. And in four years, a child has been awake a total of 16,000 hours. So figure out how many bytes that is. 10 to the 14, same number, in four years. So what that tells you is that we’re never going to get to human-level AI, which some people call AGI, but that’s a misnomer. We’re never going to get to human-level AI by just training on text. We need systems to be able to learn how the world works from sensory data. And so that means LLMs are not it. You talked about that as well. We’re not going to get to human-level AI within two years, like what some people have been saying.
Moderator: And you’ve been talking about that as well.
Dava Newman: Yeah, so that’s my point. And it’s infancy, so I think it’s actually, that’s the way to clear it. LLMs are in the infancy. A four-year-old is not an infant, but very, very early on. But when you move to generative biology, training data, when you move to sensors, the internet, when you move to the almost infinite amount of data, of information we have, and just multi-sensory. You’re talking about, you have the glasses on, your vision, but you’re looking at text. How much do we get tactile-y, hearing, sense-y, smelling, right? Have you all had your coffee this morning? What was the first thing that you really related to this morning? Probably breakfast and some coffee, smell. So when you put the multi-sensory capabilities, again, for humans, and I want to be clear from the earlier comment, humanity, flourishing humanity, and all living beings, all living, the appreciation for all of life, for all of life. Human-centered design in terms of our technologies, but you get to choose your orientation. You get to choose who you’re designing for. And so I think that’s really important, too, not the egocentric humanity versus the rest of it. You know, that’s the question, you know? How long will we be here? As I say, technology is for space up there, that’s my specialty. It doesn’t need us. You know, so a little humility, please. Being humble. Earth’s going to be fine without humanity, we’re a bit of a nuisance, a huge nuisance. So you know, Earth is 4.5 billion years old, I have my sister planet Mars, probably find past life there, about 300, 3.5 billion years old. So again, that view, let’s please, you know, with humility and approach this and then the question is, you know, do we want to live in balance? Do we want to live the best lives we can and flourish? And then I think you just approach it, you know, with different questions, you approach solutions from a different perspective.
Moderator: Thanks. I think I heard something over here. I’m not sure if it was a phone or a question. But I now I see a hand. There’s a mic coming because we have a live stream audience and say who you are.
Audience: Moritz Berlenz, Lightspeed for Deva. You know, you talk about AI, you talk about existence too, I’m glad you’re making life or working on making life, human life, a multi-planetary species. Where does AI fit in into this broader need? Do you see it as an existential threat? Do you see it as an existence enhancing technology? For example, generative bio, is it our great filter?
Dava Newman: Thank you for the question. So you know, I think we’re the threat. I think that people are the threat. You know, not my algorithms. And for, you know, the question again, when I do think about, you know, searching, finding life elsewhere in the universe, it’s a huge help. So when you say AI is not really useful anymore, it’s almost just like saying technology. So then we can get that we should say the specifics, you know, if we’re talking. So when it comes to travel space, for me, humans are here on Earth. We’re sending our probes and our scientific instruments. So it has a lot to do with autonomy and autonomous systems. The human having information here, but that loop of information sensing and exploration. But these are all autonomous robots. lots of systems. We are going to send people, and then we bring our own supercomputers with us so that first human mission to Mars will surpass our current 50 years of exploring on Mars. So that’s the benefit of humans or human intellect. So it’s a great question. So it’s a mix-up. It’s a threat, and we use it to the advantage of, again, capabilities, searching, exploring, and get, in my case, searching for the evidence of biosignatures or finding life elsewhere. So you’re focused, and you know your mission. And again, be very transparent about how you’re using algorithms, AI. And we always bring in something that’s very much missing in most of the development. When we get down to more foundational models, specific, personalized, foundational capability, whether it’s for health, or climate, or exploration, you’ve got to bring in the physics. So the physics, if you let things go just mathematically, statistically, I mean, look at where we’re at. Fantastic. But I’m a big believer. I’m a biomimicry. I’m trying to understand nature. I’m trying to understand living systems, always bringing in foundational physics with my math. And you proceed along that course.
Moderator: So while we continue the discussion in here, I also invite those online. We have a couple of questions for you. What excites you about the technology that we’re talking about? What worries you? And we have the opportunity to do some more word cloud. So if you’re online and using Slido, please share your thoughts there. And then we had a question there. They’re going to bring a microphone. Everyone, if you can just wait for a mic, it’ll help those online.
Audience: Yes, Martina Hirayama, State Secretary for Education, Research, and Innovation, Switzerland. My question goes to you, Deva. So you talk about values concerning AI. So we have a divide concerning access to AI or not. What influence will it have if we consider that we do not share the same values on Earth in all areas where we live? not even talking about space. What influence will this have on divide?
Dava Newman: I think it’s fundamental. I give a list of five or six. My hope is that we can agree on two or three of those. Just two or three of those. It probably won’t be the entire set. But I think we have to look for agreement and shared values and then work together. If not, then that’s maybe the scenario that plays out of the threat, division, destruction. I don’t want that path. I think we have an alternate path. So I think the hard work is people to people. Sure, policies, regulation, what do we agree on? What do we agree on? What future scenarios? And there are scenarios. It’s very plural. What future scenarios do we agree on? And if we can agree on some of those, if we can share some of those values. And I think we can. We can take a poll. See if we can get one amongst all this diversity here. It’s not an answer. It’s just part of the discussion of what can we share and what do we share together and make that the building blocks to get it right.
Moderator: And, Jan, that is kind of the challenge of building these systems for a globe, again, where the world doesn’t agree on a lot. There’s hopefully some basic things we agree on, though it seems like we struggle even on those. I know you’ve talked about using federated learning and to really make sure the world is represented in these models. But how do we build for a world where there is so much disagreement? Again, when AI systems aren’t going to just moderate content, they’re going to create and answer content.
Yann LeCun: Well, I think the answer to this is diversity. So, again, if you have two or three AI systems that all come from the same location, you’re not going to get diversity. So the only way to get diversity is having systems that are trained on all the languages and cultures. value systems in the world. And those are the foundation models. And then they can be fine-tuned by a large diversity of people who can build systems with different ideas of what good value systems are, and then people can choose. So it’s the same idea as a diverse press, right? You need a diversity of opinion in the press to at least have the basic ingredient of democracy. So it’s going to be the same for AI system. You need them to be diverse. So one way to do this, I mean, it’s quite possible that, it’s quite likely that it’s going to be very difficult for a single entity to train a foundation model on all the data, all the cultural data in the world. And that may eventually have to be done in sort of a federated fashion or distributed fashion where every region in the world or every interest group or whatever has their own data center and their own data set, and they contribute to training a big global model that may eventually constitute the repository of all human knowledge.
Moderator: I saw a hand over here, and if you can wait for the mic, thanks. Yeah, well, we’re past the mic. And I think
Dava Newman: that’s much more exciting to me. We’re federated, I’m talking, again, transparency, because then it’s more customized, it’s more personalized, it’s going after, you know, for the work, it’s again going after a medicine or health or a specific, you know, it can be more specific and much more precise. So to me, that’s very exciting.
Audience: Hi, my name is Mukta Joshi, and I’m from London. I was listening to a panel yesterday, and they talked about a concept that really startled me. And I went back and did a bit of research on it, and it’s called alignment faking in LLMs, which is about how, you know, the LLM models are giving answers which they are faking to align to whatever is being asked to them or whatever the general can say. It is probably an experiment that has happened in the last few months, but it was really startling, and I just thought it was really interesting. but I’d get a few thoughts from you on that.
Yann LeCun: OK. I have perhaps a slightly controversial opinion about this, which is that, to some extent, LLMs are intrinsically unsafe because they’re not controllable. You don’t really have any direct way of controlling whether what they say is certain characteristics with respect to guardrails. The only way you can do this is by training them to do it. But of course, that training can be undone by going outside of the domain where they’ve been trained. So to some extent, they are intrinsically unsafe. Now, that’s not particularly dangerous because they’re not particularly smart either, right? So they’re useful. In terms of intelligence, they are more like intelligence assistants in the sense that if they produce a text, you know that a lot of it can be wrong in it, and you have to kind of go do a pass on it and correct some of the mistakes and know what you’re doing. It’s a bit like driving assistance for cars. We don’t have completely autonomous consumer cars, but we have driving assistance, and it works pretty well. So same thing. But we should forget about LLMs. So this idea that somehow we should extrapolate the capability of LLMs and realize, oh, they can fake their intentions. First of all, they don’t have any intentions. And like, you know, stimulate values. They don’t have any values. And convince people to do horrible things. They don’t have any notion of what this is at all. And as I said, they’re not going to be with us five years from now. We’re going to have much better systems that are objective-driven, where the output that those systems will produce will be by reasoning. And the reasoning will guarantee that whatever output is produced satisfies certain guardrails, and those systems will not be able to be, it wouldn’t be possible to jailbreak them by changing the pump, basically, because that would be sort of hardwired in the guardrails.
Moderator: So given what Yann just said, Dava, You know, the big talk, the big buzz word this year is agents and giving more power to these LLMs. Given what Jan just said about their limitations, and this is one of the companies making it, should we be worried about giving more autonomy and agencies to a system that has no values, makes mistakes?
Dava Newman: Yeah. Well, and I don’t think so. I agree with what Jan said. You know, the LLMs, they’re not smart. They don’t have rationality. They don’t have an intention. I mean, they’re just lacking. Think of them as, you know, math and statistical, you know, probabilities like that. So all of the, probably what we much more care about, you know, in humans is, well, judgment. That’s what, you know, the question is like, well, this seems very alerting because it’s, you know, fakes, fakes of any types are alerting, right? So the question, what do we do about this? Because, you know, agents are, you know, agentic, you know, it is turning into agentic. So simple. There’s some simple, I don’t know if there are solutions, there’s just as simple ideas we can do, right? You know, we have copyright things and things like that. What if it just, you know, comes up every time we’re using a generative, you know, model? Why isn’t it watermarked? Why isn’t, you know, what do we know that, you know, what’s, you know, is this coming from a human? Is this, you know, coming from an algorithm? Just, you know, just visually just saying, you know, just watermark that, you know, it’s generative. Just some more information about what you’re looking at. So the person, the user, you know, if this is being, you know, served up to someone, they can take it. I want to do the flip side of this argument to debate, you know, with myself, I mean, you know, published a paper on unlocking creativity, you know, again, with machine learning. It’s fantastic. Some generative capability. You have an idea, we have an idea. So we just do some simple brainstorming and generate, again, to me, I like actually images, you know, the text because it maps to the human brain. We’re almost perfect in terms of image mapping and looking at visuals. So I’m going to say my sentence, what’s that image? And, you know, Jan can have his, and we look down and we’re going to have a really nice discussion. It’s going to help us actually be more creative, more, we can have more discussion if it’s kind of a prompt for us. You know, that’s where it’s a tool. You know, it really is then an assistant. It’s helping us converse and have a discussion or a debate. I think it should definitely be flagged. We know, we have to know where it comes from. We have to know, you know, what the ingredients are into the recipe.
Moderator: So it’s hard to believe we only have a couple minutes left and I want to give each of you a chance to give us one thing we haven’t talked about. What aren’t we talking about enough that we should be talking about and maybe we’ll be talking about next year?
Yann LeCun: Okay, I’m going to go by the list that we’ve seen here. Exactly. This is what excites you the most about technology. Brain-computer interface, forget about that. This is not happening anytime soon, at least not the invasive type that Neuralink is working on. The non-invasive type, so things like, you know, electromyogram bracelets that MITEI is working on, yes, that’s happening this year. And that’s exciting, actually. But like drilling your brain, no, except for clinical purpose. Gaming virtual world, MITEI, of course, has been sort of very active in this space with Metaverse. CIS Exploration, you are the expert. It’s exciting as well. Regulation, that’s a very interesting topic that I think people are, in government, have been brainwashed to some extent into believing in the existential risk story and has led to regulation that are, frankly, counterproductive because the effect that they have is essentially make open source, the distribution of open source AI engine, essentially illegal. And in my opinion, that’s way more dangerous than all the other potential dangers. For robotics, as I said, maybe the coming decade will be the decade of robotics because maybe we’ll have AI systems that are sufficiently smart to understand how the real world works. And in your previous cloud. There was efficiency and power consumption. Efficiency, there is enormous motivation and incentive for the industry to make AI inference more efficient. So you don’t have to worry about people not being motivated enough to make AI systems efficient. This is the main cost of running an AI system, is power consumption. So enormous amount of work there, but the technology is what it is.
Moderator: Thanks, Dave, and we have a minute left.
Dava Newman: Yeah, yeah, just beat around. I’ll take three of them. Politely disagree, brain-computer interfaces. No, it’s not, it’s not off. It’s happening now in terms of we have a digital central nervous system. So we are already having a brain control over, especially in the area of breakthrough technologies for replacement for prosthetics. So half human, half robotic. New robotic legs, you know, get rid of phantom foot because the brain is literally controlling the robot. So it’s, we’re to the cyborg phase. We’re doing that, it’s implanted. People are walking around. Soon it’ll hopefully be paraplegics. In the future, maybe quadriplegics. So the brain is controlling, you know, a digital central nervous system. The brain is quite powerful, so it’s the surgery. So I’d love to talk about that, but that’s here. That’s not even the future, that’s the now. After, you know, space, we talked about a little bit, but again, for scientific purposes, you know, finding life. What does that, why explore out in space? Because it tells us, it’s not option B. Sorry, Elon, it’s not option B. It’s for flourishing humanity. It’s to appreciate all of us together, our humanity, and what we can get right here on Earth, and definitely living in balance with Earth. But it’s necessary because when we design for space in the extreme environments of the moon, Mars, you name it, Europa, Clipper, anywhere in the solar system, exoplanets, it’s because for us, it pushes us. It pushes the technology. It makes me, you know, really sharpen the game in some of this technology. So very optimistic about that. I think we will find the evidence. of life or past life in the next decade. Robotics, this is, consumer robotics, okay, but what if it’s just the robotic, again, hardware software robotics, you think of physical systems. Well, guess what, now what robots they are. The AI, they’re the algorithms, they’re the software. So we do get to that physical cyber, we get to where we don’t talk about hardware and software, we get to just the robotics or the machine. It’s embedded with the software. My use is, my favorite use case is for health. Revolutionizing individualized, personalized medicine, things like that, rather than buying it and more stuff and more stuff and more consuming, what if you make your own? Again, we’re back to open source, let everyone do it yourself, make it yourself, open source it and use it from all recycled, let’s think about what’s circular. So what can we do with everything, any waste? That’s the new, to me, that’s the new robotic, informed physical cyber system of the future in the hands, of course, of our kids. And they’ll do some, just a little bit of education, they’ll do some pretty wonderful things with it, if you leave it to the next generation.
Moderator: Well, that’s a great place to leave things, we are gonna have to leave it there. Thank you so much, David Newman from MIT, Yann LeCun from META, everyone in the room and everyone who’s joined us.
Yann LeCun
Speech speed
162 words per minute
Speech length
3420 words
Speech time
1262 seconds
Large language models have limitations and short shelf life
Explanation
Yann LeCun argues that the current paradigm of large language models (LLMs) has a limited lifespan of about 3-5 years. He believes that within this timeframe, LLMs will be replaced by more advanced AI systems.
Evidence
LeCun states that within five years, nobody in their right mind will use LLMs anymore, at least not as the central component of an AI system.
Major Discussion Point
Current state and future of AI technology
Agreed with
– Dava Newman
Agreed on
Current AI systems have limitations
Differed with
– Dava Newman
Differed on
Future of Large Language Models (LLMs)
Need to move beyond text to sensory data and physical world understanding
Explanation
LeCun emphasizes the importance of developing AI systems that can learn from sensory data and understand the physical world. He argues that current LLMs are limited to manipulating language and lack true understanding of the real world.
Evidence
LeCun compares the amount of information processed by LLMs to that experienced by a four-year-old child, highlighting the need for AI to learn from sensory data beyond just text.
Major Discussion Point
Current state and future of AI technology
Open source and diversity are key to developing beneficial AI
Explanation
LeCun advocates for open source foundation models as a way to ensure diversity in AI development. He believes this approach will enable a wide range of AI systems that can cater to different languages, cultures, and value systems.
Evidence
LeCun suggests that open source platforms will allow for the creation of AI assistants that understand all languages, cultures, and value systems in the world.
Major Discussion Point
Ethical considerations and values in AI development
Agreed with
– Dava Newman
Agreed on
Need for human-centered AI development
Evolution of content moderation approaches at Meta
Explanation
LeCun discusses how Meta’s approach to content moderation has evolved over time. He explains that advancements in natural language understanding have improved the ability to detect problematic content automatically.
Evidence
LeCun mentions that the proportion of hate speech taken down automatically by AI systems increased from 20-25% in late 2017 to 96% in late 2022.
Major Discussion Point
Content moderation and AI’s role in society
Importance of efficiency and power consumption in AI development
Explanation
LeCun emphasizes the critical role of efficiency and power consumption in AI development. He argues that there is significant motivation within the industry to make AI inference more efficient due to the high costs associated with power consumption.
Evidence
LeCun states that power consumption is the main cost of running an AI system, driving enormous efforts to improve efficiency.
Major Discussion Point
Emerging AI applications and societal impact
Dava Newman
Speech speed
187 words per minute
Speech length
2910 words
Speech time
933 seconds
AI is still in its infancy, especially compared to human capabilities
Explanation
Dava Newman argues that current AI technologies, particularly large language models, are still in their early stages of development. She emphasizes that these systems lack the complex capabilities of human cognition and sensory processing.
Evidence
Newman compares the capabilities of current AI systems to those of very young children, highlighting the vast difference in sensory processing and understanding between AI and humans.
Major Discussion Point
Current state and future of AI technology
Agreed with
– Yann LeCun
Agreed on
Current AI systems have limitations
Differed with
– Yann LeCun
Differed on
Future of Large Language Models (LLMs)
Need for human-centered design and articulated values in AI
Explanation
Newman stresses the importance of designing AI systems with human needs and values at the forefront. She argues that developers should clearly articulate their values and ensure that AI technologies are intentionally designed for human flourishing.
Evidence
Newman lists values such as integrity, excellence, curiosity, and community as examples of what should guide AI development.
Major Discussion Point
Ethical considerations and values in AI development
Agreed with
– Yann LeCun
Agreed on
Need for human-centered AI development
Importance of transparency and trust in AI systems
Explanation
Newman emphasizes the need for transparency in AI development and deployment. She argues that users should be able to trust AI systems and understand where the training data comes from and how the systems work.
Evidence
Newman suggests implementing watermarks or other indicators to clearly identify AI-generated content and provide information about its origins.
Major Discussion Point
Ethical considerations and values in AI development
Need for better policies to address harmful content
Explanation
Newman argues that current policies for addressing harmful content online are inadequate. She emphasizes the importance of developing more effective approaches to content moderation and online safety.
Evidence
Newman states emphatically that we do not have the right policies in place, suggesting that we know what’s right and wrong in terms of human behavior and civility.
Major Discussion Point
Content moderation and AI’s role in society
Potential of brain-computer interfaces and robotics
Explanation
Newman discusses the current advancements and future potential of brain-computer interfaces and robotics. She argues that these technologies are already making significant impacts in areas such as prosthetics and medical treatments.
Evidence
Newman mentions examples of people using brain-controlled robotic prosthetics and the potential for future applications in treating conditions like paraplegia and quadriplegia.
Major Discussion Point
Emerging AI applications and societal impact
AI’s role in space exploration and finding extraterrestrial life
Explanation
Newman highlights the importance of AI in space exploration and the search for extraterrestrial life. She argues that these endeavors push technological boundaries and provide valuable insights for life on Earth.
Evidence
Newman predicts that evidence of life or past life elsewhere in the solar system will be found within the next decade, emphasizing the role of AI in this discovery.
Major Discussion Point
Emerging AI applications and societal impact
Unknown speaker
Speech speed
0 words per minute
Speech length
0 words
Speech time
1 seconds
Challenge of implementing diverse global values in AI systems
Explanation
This argument addresses the difficulty of incorporating diverse global values into AI systems. It highlights the need to consider cultural differences and varying perspectives when developing AI technologies for global use.
Major Discussion Point
Ethical considerations and values in AI development
Concern over giving more autonomy to current limited AI systems
Explanation
This argument expresses worry about granting increased autonomy to AI systems that currently have significant limitations. It suggests caution in expanding the role and decision-making power of AI given its current capabilities and potential risks.
Major Discussion Point
Ethical considerations and values in AI development
Debate over appropriate thresholds for content removal
Explanation
This argument discusses the ongoing debate about determining the right balance for content moderation on social media platforms. It involves considering factors such as free speech, user safety, and cultural sensitivities when setting thresholds for content removal.
Major Discussion Point
Content moderation and AI’s role in society
Potential for AI to enhance human creativity and discussion
Explanation
This argument suggests that AI has the potential to augment human creativity and facilitate more productive discussions. It proposes that AI tools can serve as assistants or prompts to inspire new ideas and foster deeper conversations.
Major Discussion Point
Emerging AI applications and societal impact
Concerns over AI safety and alignment
Explanation
This argument addresses worries about the safety of AI systems and their alignment with human values and intentions. It highlights the importance of ensuring that AI behaves in ways that are beneficial and not harmful to humanity.
Major Discussion Point
Ethical considerations and values in AI development
Agreements
Agreement Points
Current AI systems have limitations
speakers
– Yann LeCun
– Dava Newman
arguments
Large language models have limitations and short shelf life
AI is still in its infancy, especially compared to human capabilities
summary
Both speakers agree that current AI systems, particularly large language models, have significant limitations and are still in early stages of development compared to human capabilities.
Need for human-centered AI development
speakers
– Yann LeCun
– Dava Newman
arguments
Open source and diversity are key to developing beneficial AI
Need for human-centered design and articulated values in AI
summary
Both speakers emphasize the importance of developing AI systems that are centered around human needs, values, and diversity.
Similar Viewpoints
Both speakers advocate for more advanced AI systems that can process and understand complex sensory data, moving beyond simple text-based models. They also stress the importance of transparency in AI development.
speakers
– Yann LeCun
– Dava Newman
arguments
Need to move beyond text to sensory data and physical world understanding
Importance of transparency and trust in AI systems
Unexpected Consensus
Potential of brain-computer interfaces and robotics
speakers
– Yann LeCun
– Dava Newman
arguments
Importance of efficiency and power consumption in AI development
Potential of brain-computer interfaces and robotics
explanation
While Yann LeCun initially dismissed brain-computer interfaces as not happening soon, Dava Newman provided concrete examples of current advancements in this field. This unexpected consensus highlights the rapid progress in brain-computer interfaces and robotics, which aligns with LeCun’s emphasis on efficiency in AI development.
Overall Assessment
Summary
The main areas of agreement include the current limitations of AI systems, the need for human-centered AI development, and the importance of moving beyond text-based models to more complex sensory data processing. There is also a shared recognition of the potential in brain-computer interfaces and robotics.
Consensus level
The level of consensus among the speakers is moderate. While they agree on several fundamental points about the current state and future direction of AI, they approach these issues from different perspectives. This level of consensus suggests that there is a shared understanding of the challenges and opportunities in AI development, but diverse approaches to addressing them. This diversity of thought could lead to more comprehensive and nuanced solutions in the field of AI.
Differences
Different Viewpoints
Future of Large Language Models (LLMs)
speakers
– Yann LeCun
– Dava Newman
arguments
Large language models have limitations and short shelf life
AI is still in its infancy, especially compared to human capabilities
summary
LeCun argues that LLMs have a limited lifespan of 3-5 years, while Newman sees current AI, including LLMs, as still in its infancy compared to human capabilities.
Brain-computer interfaces
speakers
– Yann LeCun
– Dava Newman
arguments
Brain-computer interface, forget about that. This is not happening anytime soon, at least not the invasive type that Neuralink is working on.
Politely disagree, brain-computer interfaces. No, it’s not, it’s not off. It’s happening now in terms of we have a digital central nervous system.
summary
LeCun dismisses the near-term potential of invasive brain-computer interfaces, while Newman argues that such technologies are already in use and developing rapidly.
Unexpected Differences
Importance of brain-computer interfaces
speakers
– Yann LeCun
– Dava Newman
arguments
Brain-computer interface, forget about that. This is not happening anytime soon, at least not the invasive type that Neuralink is working on.
Politely disagree, brain-computer interfaces. No, it’s not, it’s not off. It’s happening now in terms of we have a digital central nervous system.
explanation
The stark difference in views on the current state and near-term potential of brain-computer interfaces is unexpected, given that both speakers are experts in technology and AI. This disagreement highlights the complexity and rapid development in this field.
Overall Assessment
summary
The main areas of disagreement revolve around the future of LLMs, the potential of brain-computer interfaces, and approaches to developing beneficial AI.
difference_level
The level of disagreement is moderate. While the speakers share some common goals, they have significant differences in their perspectives on the current state and future direction of AI technologies. These differences highlight the complexity of the field and the need for ongoing dialogue and collaboration to address the challenges and opportunities presented by AI.
Partial Agreements
Partial Agreements
Both speakers agree on the importance of developing AI that benefits humanity, but they differ in their approaches. LeCun emphasizes open source and diversity, while Newman focuses on human-centered design and clearly articulated values.
speakers
– Yann LeCun
– Dava Newman
arguments
Open source and diversity are key to developing beneficial AI
Need for human-centered design and articulated values in AI
Both speakers acknowledge the need for effective content moderation, but they differ in their assessment of current approaches. LeCun highlights improvements in AI-driven moderation, while Newman argues that current policies are inadequate.
speakers
– Yann LeCun
– Dava Newman
arguments
Evolution of content moderation approaches at Meta
Need for better policies to address harmful content
Similar Viewpoints
Both speakers advocate for more advanced AI systems that can process and understand complex sensory data, moving beyond simple text-based models. They also stress the importance of transparency in AI development.
speakers
– Yann LeCun
– Dava Newman
arguments
Need to move beyond text to sensory data and physical world understanding
Importance of transparency and trust in AI systems
Takeaways
Key Takeaways
Current AI systems like large language models have significant limitations and a likely short shelf life of 3-5 years
Future AI development needs to move beyond text to incorporate sensory data and physical world understanding
Open source and diversity are crucial for developing beneficial AI that represents global values
Human-centered design and clearly articulated values should guide AI development
Content moderation and implementing diverse global values in AI systems remain major challenges
AI has potential to enhance human creativity and capabilities in areas like space exploration, medicine, and robotics
Resolutions and Action Items
None identified
Unresolved Issues
How to effectively implement diverse global values in AI systems
Appropriate thresholds and approaches for content moderation on social platforms
Ensuring safety and alignment of increasingly autonomous AI systems
Balancing open source development with potential misuse of AI technologies
Addressing concerns over AI’s impact on employment and society
Suggested Compromises
Using federated learning to incorporate diverse global data while maintaining privacy
Implementing transparent labeling or watermarking of AI-generated content
Balancing content moderation to allow important societal debates while limiting harmful content
Focusing AI development on augmenting human capabilities rather than full autonomy
Thought Provoking Comments
LLMs are good at manipulating language, but not at thinking… We don’t know how to reproduce this [frontal cortex thinking]. So that’s what we’re working on, having systems sort of build mental models of the world.
speaker
Yann LeCun
reason
This comment provides a crucial insight into the current limitations of AI systems and the direction of future research. It challenges the hype around current AI capabilities by highlighting a fundamental gap.
impact
This shifted the discussion towards the future of AI development and the need for more advanced systems that can truly understand and reason about the world. It set up a contrast between current AI capabilities and future goals.
We’re not going to get to human-level AI within two years, like what some people have been saying.
speaker
Yann LeCun
reason
This statement directly challenges overly optimistic predictions about AI development, grounding the discussion in a more realistic timeframe.
impact
It tempered expectations about near-term AI capabilities and redirected the conversation towards the long-term challenges and goals of AI research.
Do we understand where we’re going enough to have some prediction about five years, or is it just too much wide open?
speaker
Mukesh from Bangalore, India
reason
This question from the audience cut to the heart of the uncertainty surrounding AI development and its future trajectory.
impact
It prompted a detailed response from Yann LeCun about the expected lifespan of current AI paradigms and the direction of future research, deepening the technical aspects of the discussion.
What if we get this right? So I think you really, we need to back up. So I mean, I should articulate, and in the, you know, there’s checking, what are the values? Do we have aligned values?
speaker
Dava Newman
reason
This comment shifts the focus from technical capabilities to the ethical considerations and value alignment necessary for responsible AI development.
impact
It broadened the discussion beyond technical aspects to include important considerations about values, ethics, and the societal impact of AI.
The only way to get diversity is having systems that are trained on all the languages and cultures, value systems in the world. And those are the foundation models. And then they can be fine-tuned by a large diversity of people who can build systems with different ideas of what good value systems are, and then people can choose.
speaker
Yann LeCun
reason
This comment provides a concrete approach to addressing the challenge of building AI systems that respect global diversity.
impact
It offered a potential solution to concerns about AI bias and cultural representation, steering the conversation towards practical approaches for creating more inclusive AI systems.
Overall Assessment
These key comments shaped the discussion by grounding it in the current realities of AI capabilities, challenging overly optimistic predictions, and broadening the conversation to include crucial ethical and societal considerations. The discussion evolved from technical specifics to wider implications of AI development, emphasizing the need for responsible innovation that respects diverse values and cultures. The interplay between technical limitations, future research directions, and ethical considerations provided a comprehensive view of the challenges and opportunities in AI development.
Follow-up Questions
How can we ensure open source AI models are both widely accessible and safe?
speaker
Moderator
explanation
This is important to balance the benefits of open source development with potential risks of unrestricted access to powerful AI models.
How can we develop AI systems with appropriate values and ethical frameworks for different cultural contexts?
speaker
Moderator
explanation
This is crucial for creating AI that can operate appropriately across diverse global societies with different value systems.
How will AI and other emerging technologies contribute to making human life multi-planetary?
speaker
Moritz Berlenz (audience member)
explanation
This explores the role of AI in space exploration and potential human settlement beyond Earth.
What influence will differing values across the world have on the digital divide and access to AI?
speaker
Martina Hirayama (audience member)
explanation
This addresses how cultural and value differences may impact global AI development and adoption.
How can we address the issue of ‘alignment faking’ in large language models?
speaker
Mukta Joshi (audience member)
explanation
This explores concerns about AI systems potentially giving deceptive or insincere responses to align with user expectations.
How can we develop more efficient and power-conscious AI systems?
speaker
Yann LeCun
explanation
This is important for reducing the environmental impact and operational costs of AI technologies.
What are the implications and potential applications of brain-computer interfaces?
speaker
Dava Newman
explanation
This explores the current state and future possibilities of direct neural interfaces with technology.
How can AI and robotics revolutionize personalized medicine and healthcare?
speaker
Dava Newman
explanation
This examines the potential for AI to transform medical treatments and health outcomes on an individual level.
Disclaimer: This is not an official session record. DiploAI generates these resources from audiovisual recordings, and they are presented as-is, including potential errors. Due to logistical challenges, such as discrepancies in audio/video or transcripts, names may be misspelled. We strive for accuracy to the best of our ability.
Related event
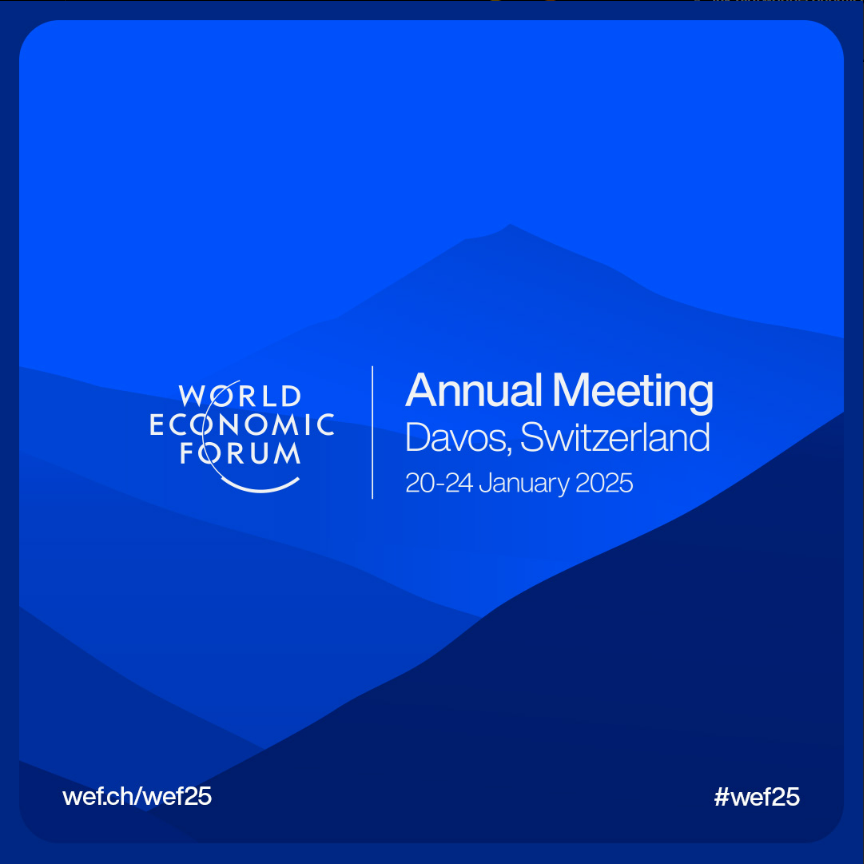