WS #31 Cybersecurity in AI: balancing innovation and risks
WS #31 Cybersecurity in AI: balancing innovation and risks
Session at a Glance
Summary
This discussion focused on the cybersecurity challenges and ethical considerations surrounding artificial intelligence (AI) systems. Experts from various fields explored the need for trust, transparency, and responsible deployment of AI technologies. They emphasized that while AI adoption is rapidly increasing across industries, concerns about security vulnerabilities and ethical implications remain.
The panelists highlighted the importance of developing comprehensive cybersecurity measures specifically tailored for AI systems. They discussed the need for guidelines and standards to help organizations implement AI securely, addressing issues like data poisoning, model security, and supply chain vulnerabilities. The experts also stressed the significance of AI literacy and education for professionals and the general public to foster responsible AI use.
The discussion touched on the challenges of harmonizing AI regulations across different jurisdictions, with some panelists suggesting that complete harmonization may not be feasible due to cultural and regional differences. However, they emphasized the importance of interoperability and common frameworks for AI governance.
Ethical considerations were a key topic, with panelists exploring the complexities of defining and implementing ethical AI practices across diverse cultural contexts. They discussed the need for balancing innovation with responsible AI development, considering factors such as fairness, transparency, and societal impact.
The experts also addressed the future of work in the context of AI, suggesting that while AI may change job roles, it is likely to create new opportunities rather than eliminate human involvement entirely. The discussion concluded by acknowledging the ongoing challenges in AI security and ethics, emphasizing the need for continued collaboration and adaptive strategies to address emerging threats and ethical dilemmas in the rapidly evolving field of AI.
Keypoints
Major discussion points:
– The importance of trust and transparency in AI systems
– Cybersecurity challenges and vulnerabilities specific to AI
– The need for AI literacy and education across society
– Ethical considerations and cultural differences in AI development and use
– Regulatory approaches and challenges in harmonizing AI governance globally
The overall purpose of the discussion was to explore key security and trust issues related to the widespread adoption of AI technologies, and to discuss potential approaches for addressing these challenges through education, guidelines, and governance frameworks.
The tone of the discussion was largely analytical and solution-oriented. Speakers approached the complex issues with a mix of caution about risks and optimism about potential benefits of AI. There was an emphasis on the need for multi-stakeholder collaboration and nuanced approaches that consider cultural and regional differences. The tone became slightly more urgent when discussing the rapid pace of AI adoption and the need to quickly develop appropriate safeguards and literacy.
Speakers
– Gladys Yiadom: Moderator
– Dr. Allison Wylde: Member of UNIGF Policy Network of Artificial Intelligence team, senior lecturer at UNIGF, assistant professor at GCU London
– Yuliya Shlychkova: Vice President of Public Affairs at Kaspersky
– Sergio Mayo Macias: Coordinator of European Digital Innovation Hub, member of IGF Policy Network of Artificial Intelligence
– Melodena Stephens: Professor of innovation and technology at Mohammed bin Rashid School of Government in Dubai, UAE
Additional speakers:
– Jochen Michels: Online moderator
– Charbel Chbeir: President of Lebanese ISOC
– Christelle Onana: Works for EODNEPAD (developing agency of the African Union)
– Francis Sitati: From Communications Authority of Kenya (regulator for ICT sector)
Full session report
Expanded Summary of AI Cybersecurity and Ethics Discussion
Introduction
This discussion, moderated by Gladys Yiadom, brought together experts from various fields to explore the cybersecurity challenges and ethical considerations surrounding artificial intelligence (AI) systems. The panel included Dr. Allison Wylde, a member of the UNIGF Policy Network of Artificial Intelligence team; Yuliya Shlychkova, Vice President of Public Affairs at Kaspersky; Sergio Mayo Macias, Coordinator of European Digital Innovation Hub; and Melodena Stephens, Professor of innovation and technology at Mohammed bin Rashid School of Government in Dubai, UAE. Additional contributors included Johan as online moderator, Charbel Chbeir from Lebanese ISOC, Christelle Onana from EODNEPAD, and Francis Sitati from the Communications Authority of Kenya.
The discussion focused on several key areas: trust and transparency in AI systems, cybersecurity challenges specific to AI, the need for AI literacy and education, ethical considerations in AI development and use, and regulatory approaches to AI governance. The overall tone was analytical and solution-oriented, with speakers balancing caution about risks with optimism about AI’s potential benefits.
Trust and AI Adoption
A central theme of the discussion was the complex nature of trust in AI systems. Allison Wylde emphasised that trust is subjective and culturally dependent, challenging the notion of universal trust standards for AI. She highlighted the difficulties in defining and measuring trust in AI systems, noting that trust varies across different contexts and cultures. Gladys Yiadom referenced a Kaspersky study indicating that over 50% of infrastructure companies have implemented AI despite trust concerns, highlighting the tension between rapid adoption and lingering scepticism.
Yuliya Shlychkova pointed out that AI, being fundamentally software, cannot be considered 100% safe, which leads to ongoing cybersecurity concerns. To address these issues, she suggested that education efforts could help build trust and harmonisation in AI adoption. This multifaceted view of trust underscored the need for nuanced approaches to fostering confidence in AI technologies.
AI Security Challenges
The discussion delved into specific cybersecurity challenges posed by AI systems. Yuliya Shlychkova highlighted vulnerabilities such as data poisoning, prompt injection, and attacks on various components of the AI development chain. She presented Kaspersky’s guidelines for AI security, which address issues like model security, supply chain vulnerabilities, and best practices for secure AI development and deployment.
Melodena Stephens raised concerns about the lack of algorithmic transparency, which makes it difficult to audit AI systems effectively. The potential security risks associated with open-source AI models were also discussed. The experts stressed the importance of developing guidelines and standards to help organisations implement AI securely, addressing issues like model security and supply chain vulnerabilities.
AI Regulation and Governance
The challenge of harmonising AI regulations globally emerged as a significant point of discussion. Dr. Alison highlighted the difficulties in achieving global harmonisation due to cultural differences, while Sergio Mayo Macias pointed to the EU AI Act as a potential model for regional AI governance. Melodena Stephens suggested that Africa has an opportunity to develop its own AI strategy and standards, reflecting the need for context-specific approaches. This was further supported by Christelle Onana, who mentioned the African Union’s continental AI strategy.
Yuliya Shlychkova emphasised the importance of self-imposed ethical standards by companies, alongside formal regulations. The discussion also touched on the role of private sector companies in AI governance and regulation. This multi-layered approach to governance reflected the complex landscape of AI development and deployment across different jurisdictions and cultural contexts.
Ethical Considerations in AI
The panel explored the complexities of defining and implementing ethical AI practices. Melodena Stephens noted that while AI ethics guidelines exist, they are often difficult to operationalise. Sergio Mayo Macias highlighted the crucial yet challenging task of ensuring algorithmic fairness and the importance of data quality in AI development. Allison Wylde emphasised how cultural norms influence the interpretation and application of ethics in AI contexts.
The discussion also touched on AI’s impact on the workforce, with Yuliya Shlychkova stressing the need for careful consideration of human-AI collaboration and the potential displacement of certain job roles. This highlighted the broader societal implications of AI adoption and the importance of balancing innovation with responsible development.
AI Education and Literacy
A consensus emerged around the critical need for increased AI literacy among professionals and the general public. Gladys Yiadom emphasised this point, while Allison Wylde highlighted the importance of youth mobilisation and education for responsible AI adoption. Melodena Stephens suggested that AI literacy efforts should distinguish between general digital skills and AI-specific knowledge, adding nuance to the discussion on education strategies.
Yuliya Shlychkova stressed the necessity of continuous training on AI risks and best practices within organisations. This focus on ongoing education reflected the rapidly evolving nature of AI technologies and the need for adaptive learning approaches.
AI in Cybersecurity
The potential use of AI in cybersecurity was discussed, with experts noting both the opportunities and challenges. While AI can enhance threat detection and response capabilities, concerns were raised about the potential for AI systems to be exploited by malicious actors. The need for robust security measures in AI-powered cybersecurity tools was emphasised.
Conclusion
The discussion concluded by acknowledging the ongoing challenges in AI security and ethics, emphasising the need for continued collaboration and adaptive strategies. Key takeaways included the subjective nature of trust in AI, the significant cybersecurity challenges faced by AI systems, the difficulties in harmonising global AI regulations, the importance of operationalising ethical guidelines, and the critical role of AI literacy.
Unresolved issues highlighted by the discussion included effective methods for harmonising AI regulations across different jurisdictions, practical implementation of AI ethics guidelines, balancing innovation with security concerns, the long-term impact of AI on the workforce, and ensuring algorithmic fairness and transparency.
The experts proposed several action items, including the use of Kaspersky’s guidelines for AI security, the development of self-imposed ethical standards by companies, and the adoption of risk-based approaches to AI regulation. The discussion underscored the complexity of global AI governance and the need for flexible, context-specific solutions that consider cultural, regional, and ethical dimensions while promoting responsible AI development and use. The importance of a multi-stakeholder approach in developing AI standards and regulations was emphasised as crucial for addressing the multifaceted challenges posed by AI technologies.
Session Transcript
Gladys Yiadom: have as recently witnessed the emergence of AI-enabled system at an incredible scale. Despite various regulatory in- Between- Yes, can you hear me now? Okay. Very good, thank you. Thank you. So I was saying a gap between the general frameworks and concrete implementation remains. We are here today with our distinguished speakers to explore which requirements should be considered and how a multi-stakeholder approach should be adopted to produce new standards for AI system. Organizations like NIST or ISO are actively developing cybersecurity standards for AI-specific threats. However, the standards mostly cover AI foundation models development or overall management of risk associated with AI. This has created a gap in AI-specific protection for organization that implements applied AI system based on existing model. My first question will be to you, Alison, but let me please first share some of your bio. Dr. Alison Wild is a member of UNIGF Policy Network of Artificial Intelligence team. In this capacity, she contributes on interoperability among AI standards, tool and practice. Previously an international commissioner on security standards, she co-shared the first standards to integrate physical and cyber security. Alison is also a senior lecturer at UNIGF. assistant professor at GCU London. She also intervenes at Cardiff University and more. My question to you, Alison, is this one. The use of AI has increased significantly worldwide in recent years. A Kaspersky studies has revealed that more than 50% of infrastructure with a third company have implemented AI and IoT in their infrastructure with a further 33% planning to adopt these interconnected technologies within two years. Does this widespread acceptance of AI mean that the issue of trust is no longer a concern for users and organizations?
Dr. Alison: Thank you, it’s a fascinating question and we’re back to trust. So thank you for inviting us here to IGF 2024. I’m delighted to be here. And I think this question of trust really follows on from earlier talks in the plenary the other day, there was Dr. Abdullah Ben-Sharif Al-Gamadi from SADIA who was talking about trust. And he said, we need to enhance trust in AI products and also to have transparency and trust. And I think this really resonates with your question. So we have the issue of people saying we want trust but the question for us is, well, what do we mean? How do we define trust? Trust is subjective. So maybe I trust you. I think I probably do. I don’t really know you too well, but I trust you. I’m a human. And so our human behavior is naturally to trust. Children trust their parents without thinking about it. And I think that’s one of the issues in business. People see a new technology and they want to be with the top technology, with the new technology. And of course they want to use it really without thinking. And I think that’s part of the issue. And of course, there’s lots more I can say about this. You know, stop me when you’ve heard enough. But I think if we look at basically how are we understanding trust? How are we defining trust? What’s our conceptual framework for trust? What’s your trust in your culture? Are you a high trusting nation or not, depending on where you are in the world? So we need to really look at this as a subjective issue and start with that. So I can come back again, but maybe if I can, a few more things. So I think because trust is subjective, we can’t use statistics. We can’t use regression. We can’t go with central tendency. This is not something we can run a regression model and look at, I don’t know, cultural trust measures and look across the world. We can’t do that because it’s subjective. So we need to have something more sophisticated if we’re going to really try and get the conception right and then ideally get towards some sorts of measurements. So if prominent members are calling for trust, then well, what do they mean? And how are we going to have a conceptual framework for that and how are we going to measure it and how are we going to implement it if we don’t know what we’re talking about? Now, thank you. I’ll hand over. Thank you.
Gladys Yiadom: Thank you very much, Alison, for those points as your highlighted trust is a key element here. So I’ll hand it over now to Yulia, but before asking my question, Yulia Shishkova serves as Vice President of Public Affairs at Kaspersky. She leads the company relation with government agencies, international organization and other stakeholders. She oversees Kaspersky participation in public consultation at regional and national level on key topics such as artificial intelligence, everything related to AI ethics and also governance. My question to you, Yulia is, if there are still concerns regarding the trustworthiness of AI, what are the main reasons for this mistrust? Could you give us a brief overview of the current cyber threat landscape in relation to AI?
Yuliya Shlychkova: Sure. So I am represented in a cybersecurity company and our experts do research on threats. And we actually see that AI is still software and software is not 100% safe and protected. Therefore, there are already registered cases of AI being used by cyber criminals in designing their attacks and also AI has been attacked. So that’s why people with understanding of the matters do have concerns. And this is also only cyber security angle because AI also brings a lot of sociological, social concerns, ESG concerns. But if we back to cyber security area. So we actually see that more and more cyber criminals trying to automate their routine tasks using AI. So there are a lot of talks on the dark webs, them sharing like how to automate this and that. Also on the dark web, they are trying to sell hacked chat GPT accounts and those are trading very high. So we are also being attacked. Some of the examples of attacks include data poisoning, like open source data sets used to train models. We saw backdoors and vulnerabilities there. Also, so such attacks in the wild as prompt injection when attack is targeting the algorithm, how AI model works and trying to impact the output of the model. And what’s happening like because so many organizations like to play with AI. And Gladys mentioned this way Kaspersky did, but those people who were answering how many organizations using AI, they don’t even know the scale of shadow AI use in the organizations because a lot of employees. are reaching chat GPT to do their regular work quickly. So there is an absence of knowledge like how many of these services are used. And what is happening is that employees are sharing confidential business information, financial information with AI models and those models can be impacted and this information can get into wrong hands. So just to summarize that we almost see in the wild attacks on every component of AI development chain. Therefore, cybersecurity should be addressed. We need to talk about this and help not to stop AI usage but to do it safely and have basis for this trust in for AI use in the organization.
Gladys Yiadom: Thank you. Thank you, Yulia for this comment mentioning the use of AI and the idea that we need to be careful in terms of models. It leads me to the question that I will now address to Sergio, but before my question, Sergio Mayo has more than 20 years of innovation program and information system management in various fields such as finance, telecommunication, health and more. He cooperate with IGF as a member of the Policy Network of Artificial Intelligence as a member of it since 2023. He focuses on the social impact of AI and data technologies and digital ethnography. He currently coordinates the European Digital Innovation Hub. So Sergio, thank you very much for being with us today online. My question to you, given that the internet contains a wealth of information, sometimes contradictory or even fake, can one rely on the datasets utilized to train AI models?
Sergio Mayo Macias: Good morning. Good morning. Thank you. Thank you, Gladys. you to the organization for inviting me to this workshop. Well, actually, I think that trusting the data used to train AI models is part trusting the technology and part trusting in the human creating or operating that technology. And that’s a philosophy question. I will not go deeper in this, but going deeper regarding the data issues for trusting or not trusting in data used for training AI, there are an amount of problems really, really big. And I will mention some of them. First of all, and the most important one that comes to our mind is data bias. Data bias, of course, when the training data used to develop AI models is not representative for or of the real world scenario that it is intended to model. And if the data is skewed in terms of gender, ethnicity, location, or any other attributes, the AI model will inherit and amplify these biases. And this can result in unfair predictions, discrimination, and so on. But also, even though we have the data quality issues, like poor quality data, which includes incomplete or outdated information, and it also can severely undermine the reliability of AI models. But at the end of the day, even if we have a good data set, we have a human using this data, and a human creating an algorithm and a model. So going beyond the good or bad data that we used for training this model, we have to put the focus on the algorithmic fairness. And the algorithmic fairness is is an issue that is directly pointed at the human using the data. So the human using the data must be aware of the quality of this data, must be avoid the data bias, the data privacy concerns, for instance, and so on, the data manipulation, the insufficient data representation. But at the end of the day, he’s able to produce a fair algorithm with this data. So I think this is the key point for this question.
Gladys Yiadom: Thank you. Thank you, Sergio, for your comments. So now I will turn over Melodina. Melodina is a professor of innovation and technology at Mohammed bin Rashid School of Government in Dubai, UAE. She has three decades of senior leadership international experience and consult with organizations such as Agile Nation, Council of Europe, and the Dubai Future Foundation. So we were previously addressing regulatory issues. My question to you, to maintain the balance between the progress and the security, it is assumed that the emergence of new technology should be accompanied by the development of a corresponding regulatory base. Can we say that the current governance of AI is adequate? Are existing standards such as ISO or NIST sufficient for the security of AI? Or do we need specific regulations?
Melodena Stephens: So thank you for the question. I think it’s a complex one. So let me start from the top. If you look at how many policies are there for cybersecurity, I think there are more than 100 countries which have policies. While some of them are on security and they’re looking at algorithmic security, we see recently over the last two years maybe more focusing on critical infrastructure. And there’s two things driving it. One is we’re moving away from individual security. or corporate security or industry security to national security. So this becomes an interesting trend, right? And I think the main thing, the challenge we have is fragmentation. AI is global. If you just look at the supply chain of AI, it is impossible to nationalize it. So how can you maintain even national security or individual security or corporate security when AI is global? So that’s the first thing, fragmented regulations. Anu Bradford has written an interesting book that’s called Atlas of AI, and she divides the world into three. On one end, she looks at US as a very market-focused leadership. So you see private tech actually leading and dominating. If you look at US and its allies, I think we’re talking about 27 countries if you’d look at NATO alliance. Then she looks at the EU, which she says is driven by human rights and rule of law and democracy. Again, 27 countries if you look at it. And then she talks about state-driven national strategies, and you’re looking at countries like China. If I just take the BRI project, you’re talking about approximately 140 countries. So then you’ve got a good idea of how this fragmentation and how alliances will be created across the world. So it’s very geopolitical. If I look at the strategies that are currently, or the frameworks that you mentioned, the ISO and the NIST, so there are a couple of challenges with it. One, the scope and context is decided by the organization itself. So it’s not really taking the wider perspective. And we see in strategies like this, we need whole of society, whole of government, and whole of industry perspectives, which are missing, right? And I think also the focus on risks is a challenge itself. Because when you come to a place like cybersecurity, you’re looking at a public value domain space. And it’s really about decisions on trade-offs. Do I put national security ahead of individual privacy? That’s a trade-off. Do I invest in today’s technology knowing that a data center costs billions, right? And I know that it will create an environmental footprint and a sustainability issue later. That’s a trade-off. Do I connect everything through the internet of things, which is great, but that means I am creating vulnerabilities because of all of these connections because no one company has the technology stack from bottom to the end. So that’s a trade-off. I do not think when we talk of risks, we talk enough about trade-offs and that’s one of my concerns.
Gladys Yiadom: Absolutely right, Melodina. And I think we’ll also dive into it a bit later in the session. I also invite afterwards participants to share any question that they would have. So now moving to that, this workshop is also the opportunity to display some of the guidelines that has been produced with Kaspersky team, but also the speakers that are here among us. So I’ll kindly ask the team to share the slides. Yeah, can we please share the slide and the floor will be yours, Julia.
Yuliya Shlychkova: So while we are waiting for the slides. Thank you. Okay, so as Melodina said, a lot of focus is on critical use of AI and on developers of large language models on like national competitiveness in the area of AI. And we see that there is this gap because adoption of AI is happening on the mass. scale and it’s skyrocketing. And these users, these organizations who are fine-tuning existing models and using it also need some sort of guidance. Maybe not regulation, not compliance, not requirements, but at least some guidance. Do these 10 things and you will be at least 80% more secure. And this is what we have put our thoughts into and produced these guidelines. Just a little bit to illustrate the scale of adoption, that more than a million models are available in the public repository. And like developers at GitHub are already saying that the majority of them, they are using AI at some point and industries. So in a few years, I think there will be no one not using this. Attacks I already covered in my short intervention, but again, we see almost every point in AI supply chain can be vulnerable to attacks. In public, we see more than 500 records of vulnerabilities in AI and their accounting. So we asked in our survey, professionals working in organizations, do they estimate the rise or decrease of incidents within their organization? And the majority, more than 70% reported they see a rise in such incidents. But interesting thing that 46% out of these believe that these attacks were with AI use in that way or another. And also the same professionals also reported that they believe they are not equipped enough to address these challenges. They have lack of training, lack of qualified staff, insufficient IT team size. So these problems already here, they already exist. And when we add AI usage, especially shadow usage, so it’s like with immune system, every person has, right? So it breaks under pressure. So that’s why we believe some guidance, some basic requirements are of help to organizations adopting AI. So our guidelines cover four main pillars, key security foundations, infrastructure and data protection requirements, how can resilience achieve through validation and testing, and also adherence to governance and compliance. So talking about AI security foundations, we believe that first of all, leadership organization has to know about what AI services are used and whether they open new threats or not and how those are mitigated. Team has to be trained. IT professionals has to be trained on AI usage and risk associated, and also regular users who can use AI in their work also needs to have this awareness about risks and what to do and what not to do. And these courses has to be regularly updated. There needs to be field exercises and it should be continuous exercise. Also, the response of organization has to be proportional to the use. So each organization is advised to have threats modeling about what, check, check, what threats of non-using AI can be, what threats of misusing AI can be, and how those different threats can be addressed. So to have individual threats modeling is very recommended. Talking about infrastructure security, a lot of organizations are relying on cloud-based services, hence traditional approach to infrastructure security is also relevant here. That access to AI services has to be very, has to be locked, has to be limited only to those employees who need to have this access. They have to be two-factor authentication, there has to be segmentation like data models in one place, weights in another place. So it’s all mentioned in our guidelines and I will provide you link further just mentioning highlights of this. Then talking about supply chain, in a lot of regions some AI models, popular models are not available. That’s why a lot of organizations turn into proxies, some third parties and some of them can be reliable and some not. That’s why it’s very important to check from which source information coming and to have this audit of supply chain. Because of this, a lot of organizations also choose to have localized data models within the organization and if you choose this approach, there is also importance to follow requirements such as login access, keeping and backing up your assets. Then if your use is very wide of AI within an organization, you need to be prepared against machine learning specific attacks and there are already best practices how to do this. You see fancy words like distillation techniques, train models with adversarial examples. Like for policy people like this, it might sound as rocket science but IT people would know what this means. and we provide more details in our guidelines. Then also Sergio mentioned that if you’re using a model from a third party, this model was trained on specific examples, specific data sets. So before releasing it to public, you need to train this on the real life scenarios, on your industry benchmarks in real life. So testing and validation is really important, and you need to be ready to back up to the previous version if testing goes wrong. And also general cyber security requirements. Please ensure to have regular security updates when you monitor in public sources information about vulnerabilities. Have internal audits regularly to test and update based on this test. And of course, vulnerability and bias reporting. As an organization, you need to have information available to public so that users and your clients using your AI services have an opportunity to contact you if they notice vulnerability or bias, and you have an opportunity to fine tune this. And we also as an organization, very advocate for public bug bounties programs to include AI in your bug bounty programs to have more and more community engaged. Check, check. I’m speaking too long. So vulnerability reporting is important. And of course, since regulatory space is very, very active, it’s important to keep an eye and ensure that what you are using is adhering to the standards and regulation. And I think the last slide is the most important So the full text can be accessible upon this link It’s over 10 pages We really did our best and a big thank you to Alison, Melodyne and Sergio in reviewing it and contributing And the idea of these basic standards actually come from cyber security A lot of nations like UK, Germany and ministries of communications and technologies are trying to raise awareness of these basic cyber security standards and publish this information on their website So we believe that it would be a good idea if nations worldwide can also maybe take a look at what we have produced develop, fine tune it and to promote it on national and international level so that mass usage of AI can happen in a more secure way Thank you for the opportunity
Gladys Yiadom: Thank you very much, Julia, for sharing the guidelines Again, do not hesitate also to pass the Kaspersky book if you don’t get the chance to download it here So now, moving to another set of questions Julia, you were mentioning somehow AI trainings, literacy and my question will be to you, Melodyne In such cases, how best to address the issue of increasing AI literacy among professionals but also the wider population?
Melodena Stephens: Thank you First of all, I want to mention that digital literacy is not the same thing as AI literacy So I was having a conversation Some key places people think it falls under but right now most of what passes for digital literacy is actually digital skills training, and I don’t think it’s the same thing. So we need to be very mindful of that. AI is a much more complicated topic. And I think the challenge that we’re really facing is we need societal education, we need education of industry, we need education of policy makers. I have met engineers, I work with IEEE for example, even engineers struggle when you look at AI and you look at some of how it’s being deployed or what implications it has. So this becomes a challenge. And when you look at some of the policies, I just wanna take an example. If I look at NIST, there’s 108 subcategories. If I look at ISO, for example, we’re talking about 93 controls. And what people are doing is making them 93 policies. I don’t know about you, I don’t know who reads 93 policies, but the problem is actually operationalizing it and implementing it. So the way we’re delivering knowledge, the current method is not working. An audit system, the policies put over there, we don’t know how to translate it, we don’t know what it means for me. So we need to be able to translate this for different people based on their level of expertise. And I’ll just give you one example. I heard the word, you mentioned transparency. How can we get algorithmic transparency? If I look at what Google has just released in the last week, which is Willow, it does a calculation in five minutes, which according to them, a supercomputer will take 10 raised to 25 years. That’s 10 septillion years to do. Which human being can go and look at this and trace everything? It is impossible at the speed at which technology is doing. Just another example, if you’re talking about 175 billion parameters, we’re talking about 10 million queries per day. How many people do you have to employ to go and audit 10 million queries per day? So what we’re doing right now is taking a rough sample and we’re auditing it and then we’re reporting error rates and we’re only reporting sometimes one. one type, not false negatives, not false positives. Both are important. So there’s a lot of things that are missing currently right now in the way we’re evaluating AI. And I wanna also highlight something like this because they talk about, let’s have human in the loop. If anyone has read the foreign policy article on Lavender, Project Lavender, which was a facial recognition drone technology, they did have humans in the loop to decide who or what to target. The amount of time they spent, 20 seconds for review. I don’t know about you, but my brain does not think in 20 seconds of review. We’re not computers. So the first thing is I’m not a machine, I’m a human being. My skills are different from a machine. We need to understand both of that. And I think AI literacy is kind of understanding what a machine can do, what a machine cannot do. And I’ll take the last example, which was in 2021, Facebook had an outage. It was a BGP, Border Control Gateway, Border Gateway Protocol issue. Now, what was interesting is they’re very high tech. So their systems are all on facial recognition and authentication. So they should have been able to enter in to fix the issue. Unfortunately, what happened is they got locked out of their own offices. So you have backups and we’re depending on technology for those backups, but at the end, it’s the human being. So you’ve got to have a backup, which is a human being. And my worry right now is the knowledge those human beings are having are becoming obsolete because we’re not valuing it enough.
Gladys Yiadom: Thank you. Thank you, Melodina, for this comment. My next question to you, Alison, how can a zero trust approach be integrated into the development and use of AI?
Dr. Alison: Thank you, I’m just checking. That’s great, thank you. So just very quick, Zero Trust 101, I’m sure you’re all familiar, but for those of you that are not. So as I mentioned before, we’re humans, so we’re predisposed to. presumptive trust, to trust someone without validating. I think my Russian’s really bad. So trust but verify and of course now we don’t trust, we have to verify first. So zero trust, non-presumptive trust, we have to verify an identity whether it’s an individual, a person, a data user, a technology and so on or an application. We have to verify that before we can grant trust. So we have continuous monitoring. So in a process like artificial intelligence where we’re looking across a very complex dynamic ecosystem, we’ve got all of the moving parts all moving at the same moment, the humans taking decisions, the prompts going in, the black box doing its thing with the model we’re not sure where it’s come from, the data we’re using to train the input, the outputs coming out. So we’re saying operate zero trust throughout this ecosystem to give us a chance to verify before things come out the other side and before they’re implemented and as we’ve said, colleagues have said, companies are just doing this without thinking, just like a new technology, just like driving a car before people had a driving license, jump in the car and drive in and people don’t know what they’re doing. Same in industry at the moment. Industry’s adopting this at pace and at scale without, I think the word is guardrails and zero trust can be one of the guardrails. I’m happy to come back in more depth and questions later on. I think interoperability I think is the other thing for zero trust because we’ve got everything happening at the same time at scale with no common frameworks from whether it’s our friends in ESO or NIST or wherever in the corporate world, using technology, developing standards with no interoperability across those different domains. So it’s a very complicated systems-based ecosystem.
Gladys Yiadom: And basically what you’re saying is about how to use it responsibly. So it will lead me to my next question to you, Sergio. Given your experience as a coordinator of a regional European digital innovation hub, could you please tell us more about blueprints of best practices for the responsible deployment of AI in Europe?
Sergio Mayo Macias: Yes, thank you. Thank you, Gladys. Well, actually, the AI environment in Europe is known and has been, let’s say, labelled as a regulation-focused environment. This is because the AI Act and the DATA Act, among many others, as the main European general outcomes or the known reference frameworks, but this is only partly true. The bottom-up work has been going on for a long time. I always put the same example. We don’t have a Boeing company in Europe, we don’t have this US big company, but we have Airbus, which is not a big company, but a consortia of really, really small companies. So the way we are working in Europe is this way, the cooperation, the consortium, and so on. For instance, from 2099, there is a group called the High-Level Expert Group on Artificial Intelligence, established by the European Commission, and they, in 2019, they provided the ethics and guidelines for trustworthy AI. These guidelines emphasise the need for AI systems to be lawful, ethical, and robust, and they are producing, year after year, new drafts regarding this regulation. But we also have the AI office. supporting the development and the use of trustworthy AI. And this is only from the top, but we are also working from the bottom, from small companies and organization and RTOs. And for instance, in January, 2024, the commission launched an AI innovation package called the Gen AI for EU Initiative, which is a really easy reading package to support the startups and SMEs in developing trustworthy AI that complies with EU values. So all these islands are intended and are developed for providing security by default. Let’s say for SMEs and citizens not being able to be aware of the law, to be aware of the AI Act and so on. Another initiative is the Data Spaces Support Center. This Data Spaces Support Center was launched for contributing to the creation of common data spaces. Data spaces are a safe space for collectively create a data sovereignty, interoperable and trustworthy data sharing environment. And they are directly related to the AI deployment. They point to the core issue, the creation of trust. As Alison said, if you can create an environment where data is safe, reliable and secure, you are enhancing trust. And from there, you can go a step farther and use this data for training AI models. And also the network of European Digital Innovation Hubs, I am the coordinator of the one in Aragon region in Spain. We are close to the city. We are producing guidelines, blueprints and a lot of help for this key issue to create security and trust by default and letting people using AI not being aware of big documents or big frameworks or the act or the data act.
Gladys Yiadom: Thank you. Thank you, Sergio. Mentioning regulations and just coming back also to what you said, Alison, about interoperability. Is there a need to harmonize AI regulation from different jurisdictions? If so, is it possible to ensure such interoperability?
Dr. Alison: Thank you. So two parts. The first is, is it a requirement and is there a need? Is that correct? Sorry. Sorry.
Gladys Yiadom: Yes. Let me repeat that question. So is there a need to harmonize AI regulations from different jurisdictions? And if so, is it possible to ensure such interoperability?
Dr. Alison: Okay. Thank you. So I speak from a personal perspective here. So I don’t know if, realistically, I don’t know if harmonization’s possible because we’re looking across the world, across multi-stakeholder groups, private sector, governments, state actors, individuals. And it’s really difficult because there’s different cultures in play. And I think it’s right that individuals should have their culture and should have their way of being. So I really, I think that’s really hard. I think for cybersecurity and risk management standards, we do see some global take-up of the big standards there. So maybe we can look to what’s happened with those iso20s. 27,000, 27,001 family, or even the 9,001, the kind of quality management standards there. And look at what’s happened there as a guide to what might happen in the future. But I think there will always be difference. Differences across the globe, across private sector, different sectors. So I don’t actually know, this is my personal view, if harmonization is possible. Is it desirable in an ideal world, we would have interoperability across tools, across standards and frameworks, across all of those different factors, that would be the ideal. Whether it’s possible, I don’t know. But I certainly think guidelines are really a helpful stepping stone forward. So if everyone has the same framework to work from, and a common understanding, I think that’s a really big step in trying to achieve a future where we all understand where we’re going. If that, I hope that answers your question.
Gladys Yiadom: Thank you. Absolutely, Alice, and thank you. Thank you very much. My next question will be to Yulia and Sergio. Yulia, you mentioned how important it was to address it from a cybersecurity perspective. Why is the issue of cybersecurity crucial for AI systems? What would be state-of-the-art security for AI system look like?
Yuliya Shlychkova: So we believe that’s… It’s not working. Check, check. No. Check, check, check. Check, check, check. Yes, working. Now it’s working. Okay. We can hear you. So with AI is a new thing. So every technology first developing, and then people have this afterthought, oh, I had to put more thoughts about security there. So with AI, we have this opportunity to think about security by design. The same as regulation. Like regulation is always catching up. With AI, hopefully, there is a chance not to be a decade late That’s why it’s important to keep on par and think about cybersecurity Not only about how technologically to protect this, but also to spread awareness about issues so that regular users are not feeding AI with their personal data without necessity. Employees don’t share confidential information, etc. Sergio, do you want to add?
Sergio Mayo Macias: Yes, I agree with you. I think that for AI, we cannot push people to install the antivirus That is not realistic. We need to provide cybersecurity by default We cannot send the elephant in the room to final users. We have to define safe spaces for using the AI systems and we cannot expect final users to do it. For instance, I was mentioning before the data spaces pursue that goal. To create this framework, a space with legal governance and also technical issues are developed and deployed by default, just to be used So, we have the AI Act in the background, but we have to define these spaces for letting users use AI without concerning any other issue
Gladys Yiadom: Thank you, Sergio. Perhaps, turning to the audience to check if there are any questions Yes, we do have one question here. Sir, can we ask you to come to the middle and ask your question So, please share your name, organization and who you address the question to
AUDIENCE: Yeah, so my question is actually from Yulia, as she mentioned, you know, so there has been very difference in the conventional security and the AI security. For example, in conventional security, if you send certain requests, you get the same responses. In AI, it’s very different. So I mean, how do you see the security if every time the response generated is different? I mean, if even you train your model, you cannot expect if it will provide the same answer next time. You know, so like we are actually a security firm and we work heavily in the AI security right now. So we have faced these problems, like I mean, the security options which we provide to our clients. Even if you try after sometimes, the same errors, the same vulnerabilities arises again. You cannot handle it properly. So number one, how do you see that? As far as the vulnerability disclosure program you mentioned, I mean, companies are not taking it seriously. For example, if you report biasness as a vulnerability or as an issue, they’re not accepting. Even if you see the bug bounty program of the open AI and the bug crowd, they have clearly mentioned we are not accepting like biasness or racial or unethical responses in the report. So how do you see that? I would love to see the response on that if you, yeah, thank you.
Yuliya Shlychkova: So I think I like your comments and so I think they’re more like comments than questions. Thank you for sharing your experiences and for bug bounty, it took years for big companies to start doing bug bounties and vulnerability reporting. So I think that we, you, us, we just need to push for it and do this awareness. I’m sorry, we are human beings. It takes a while for us to accept the problem and start moving to the solution. As for the issue with the AI security being different, we also see this, we are using machine learning in our solutions for ages. And again, you need to ensure that you have representative data sets to train your model Then you’re dealing with these false positives, false negatives Like trying to find the bar where the performance is okay and acceptable But still, we have this human control on the top Because 100% confidence is not there That’s why we have human experts who are analysing the output and can interfere So what we call it, it’s multi-layered protection So we are trying to use different models, they’re checking on each other And at the end of the pyramid, there is human factor
Gladys Yiadom: Thank you, Yulia, for your response I’ll just take one online question and then I’ll hand it over to you So I believe, Johan, we do have one question online
Lufunu Chikalanga: More than one question Actually, there are three questions First of all, it was valued very much that the report was shared And also, there was positive feedback to Alan’s remarks with regard to trust must verify And having that transparency aspect with regard to cyber security and artificial intelligence One question to Yulia Please excuse if I misspell your name Lufunu Chikalanga from Osis Orisur Consulting He is interested to get some information about the role of open source and artificial intelligence And in particular, he raised the question whether it is enhancing security or increasing vulnerabilities
Yuliya Shlychkova: It’s a very good question So, on one side, we advocate for open source and it’s great that community being built around AI, models being shared, data sets being shared because it’s not, it’s limited innovation if it’s only proprietary models. So, and especially for regions like Africa and others, I think it gives opportunity to leverage innovation, like this openness, availability for open source information. On the other side, those who are deploying the models needs to own responsibility of security for the things they are using and to check, to audit, to do not admit that if someone developed this for you, it’s like 100% ideal. So, this would be my answer. Please, our panelists, add on to this.
Gladys Yiadom: Do you have any other comments from our panelists, perhaps on this topic?
AUDIENCE: Yeah, I like open source, but I would jump in and say, I think there is a role for closed source. I think it’s perfectly valid, for example, if you’re using AI for cybersecurity and that goes back to a question over here. I think it’s really good to have transparency, to know what you’re using as the training data. But yes, there’s the issue of innovation. I’m sure in the future, there’ll be a way beyond this. So, having a closed system that’s off the cloud, that’s proprietary, that’s able to learn and has that security badge.
Yuliya Shlychkova: I want to have something in the middle because we as a company, we do have transparency centers where in the secure environment, we are sharing the models we’re using, our data processing principles. So, this can be shared, but in a secured environment. Yeah, good point.
Gladys Yiadom: Thank you, Yulia. So, perhaps before taking another question online, Johan, we have one question in the audience here. Can we ask the person to answer?
AUDIENCE: Thank you. Sorry, do you hear me? Yes, we can hear you perfectly. Thank you very much for the panel. It’s very interesting. But I have a question maybe for Julian. I mean, the issue, I mean, when we speak about AI and security, okay, we have AI that could be used for enhancing security. We have the normal security issue about platform infrastructure data, data center, blah, blah, blah. And then we have the data security. I mean, when we speak, if there is any other dimension that we miss, I mean, there is in the algorithmic, in addition to these ones, I mean, because whenever, I mean, I have the feeling that it’s more data security and infrastructure security at large. But there is anything related to, let us say, machine learning process or algorithmic process that we have to consider according to your knowledge on this regard? I’m not sure it’s clear, but I have the feeling that we mix AI security with data security and infrastructure security. Is there is any other dimension? Model?
Yuliya Shlychkova: I have this headset. That’s why I feel that it’s also working as a mic. I believe that you’re right, that model security is also, should be considered in the holistic picture because this is black box and we can be in classic programming to be sure that the code will perform as intended. Therefore, it’s very important to test model. And we already saw adversarial attacks trying to impact the way how model functions, maybe to add noise and invisible for AI and let model misperform. So model security is also in the question, the algorithm. Definitely.
AUDIENCE: So I was just going to add today about, if you look at the traffic on the internet, 70 to 80% is API calls, which basically means it’s code talking to code. And each one of that is a vulnerability. So it’s not just data and critical infrastructure. I think it is also because we’re looking at algorithms which are made with different languages and we’re trying to map them together with interoperability and it is not working. So one update is happening. We’re not updating in real time. And I saw a piece of research that says it takes about 200 days on an average to find a security vulnerability. That’s 200 days for a hacker to access your data. So just think of all of us. We’re here at a conference. How many of you have ensured that your data and your devices are updated? And that’s the challenge, right? Yeah.
Dr. Alison: Thank you. I’ll jump in really quickly. I think some developers are like chefs. They have their cuisine and they use their process for the model and your mother’s process is probably different from mine. So I think there’s probably a lack of, what’s the word? Replicability in the model of who’s designed it and passing the steps to the next person. And once the model starts going, then we don’t know what’s happening and there’s no record. Thanks.
Gladys Yiadom: Sergio, do you have any comments?
Sergio Mayo Macias: Yes, please. Yes, indeed, indeed. I’m really happy to hear this question and I totally agree with Melody and Alison’s comments. And let’s say that we have an ideal world with no data problems and we have fair data, secure data, reliability data, and so on, so on. And data is not problem anymore. This is an ideal world. This is impossible at all, but let’s think about that. Afterwards. As you said, there is a programmer. We have the black box. We have the algorithm. And we have the human being there, using fair data, good data, data with no problems, with no bias, and so on. And what do we do with the black box? It is the same that happened, if you remember, with COVID crisis, with the vaccine. We have the chemistry and so on. The chemistry is data. The components. But afterwards, we have the people working with those components. Let’s say the programmers here with the black box. Do we trust them? And as I already said, at the end of the day, trust is not about data. It’s trust about human beings. So we have going beyond trusting data. We have to go beyond trusting the black box. We have to think about if we are ready to trust in human beings and developing their models.
Gladys Yiadom: Thank you, Sergio. Almost a philosophical question, right? At the end of the day. Yes. Indeed. The key in this. Thank you. Johan, do we have another question online, please?
Jochen Michels: Yes, we have. Some of them were partly answered by Sergio, for example. But I will first share the question. One question is by Max Kevin Belly there. He would like to know what is the relationship between regional legislation and limitations with regard to artificial intelligence and also on… on the level of different states, and whether that is a hurdle to try to find harmonized rules and harmonized, global harmonized regulations in that regard. So some standards, that is a question perhaps to Melodina and Sergio, and there is one further question by Maha Ahmad, and that was also particularly answered by Sergio, it’s about classification of AI technology, and Sergio already referred to the European AI Act and the risk-based approach, but perhaps Alison or Melodina, perhaps you can share examples from other regions, whether there is the same approach or whether there is another approach regarding high-risk AI, low-risk AI, and so forth. Thank you. That were the questions here from the online attendees.
Gladys Yiadom: Thank you, Johan. So perhaps Melodina.
Melodena Stephens: Okay, so the first question. The first question was on AI regulations and regionalization. Okay, so the EU is the only one that I would look at it currently right now that has…
Gladys Yiadom: No, it’s good. It’s working.
Melodena Stephens: Harmonized across its 27 countries, but we also see that it is in implementation, right? So it will take some time, and right now what we don’t have is time. With the rest of the world, what I’m seeing is a strong trend towards bilateral agreements, and part of it is on defense, part of it is on data sharing, and another big one on knowledge and talent. So we’re seeing a slightly, so much more polarized world where it’s focusing on bilateral ties, and this becomes very interesting. If you want to take a step further, is it about governments? Is it about tech firms? I think that is a far more interesting discussion for me. If I look at the 500 cables that are undersea that are transmitting about 99… 99% of the data, most of them have private ownership. If I see data centers, most of them are again private. So I think there’s a whole other discussion which we are not taking into place in policy regulations, which is the role of private sector, many of which these companies are having revenues and market capitalizations much larger than countries. So you can see a power asymmetry coming in over there. I think the second question was on… Classification right here. So I know this is an interesting one. So besides risk, I’m gonna move away from risk. There’s been a lot of debate on whether we should look at it as AI technologies or AI for industry regulations. And this is a hard one because what we’re seeing right now, if I ask you a question, is Tesla a car with software or is it software disguised as a car? What do you think it is? And therefore, how should it be regulated? And the very fact, if we don’t have an answer tells… But the fact it’s… Sorry, he says software.
Charbel Shbir: Hello. Yes, it is. Hello, my name is Charbel Shbir. I’m president of Lebanese ISOC. Regarding your question, I think it’s a software developed by a person or a developer engineer. So therefore, the regulation must be… He has liability regarding the software that he developed. This is my answer. It’s not about the car as it’s a car, because it is autonomous and it’s worked by itself. Reason why he should hold the responsibility because he developed the software. But I have another intervention.
Gladys Yiadom: But I just wanna add one point. You’re right, but when it is registered, how is it registered?
Charbel Shbir: It will be registered as a car. It is registered as a car, but the responsibility is who’s driving the car.
Melodena Stephens: That is why there are challenges. So think of your health app. Apple, is it a watch or is it a health app, right? And I think this is where we’re gonna have these interesting discussions on jurisdiction that AI will move across industries and we don’t have oversight. So the purpose with which it was developed for one purpose allows it to scale into a totally different industry for another purpose and we don’t have transparency on weights, why were those weights developed? It was developed for health, but now it’s being used in X case. And I think that’s the challenge. So thank you, thank you for that answer.
Gladys Yiadom: Thank you, and Melodina, perhaps ask Sergio if you have any further comments regarding the first question that was asked and then I will hand it over to Alison.
Sergio Mayo Macias: Well, actually, yes, it’s just more or less repeating the same that you said, but also I agree with Melodina that regarding data, being able to establish contracts for ensuring trust is the key issue now. Now with data spaces in European Union, we are trying to skip that problem for SMEs and for citizens and to establish this safe space with no need of contracts, with no need of agreements for sharing data. And actually I am aware that this model is being, is let’s say also used in some countries in Latin America. They are consulting us on why we are doing these data spaces and how they work. And they are trying to do more or less the same in South America for sharing data without the need of establishing this type of one contract or one agreement for each time that we share data.
Gladys Yiadom: Thank you, Sergio. Alison, please.
Dr. Alison: Thank you, just to jump back in. So the question of high risk contexts. So I was at Warwick University a couple of weeks ago with some of the MSc students coming in from industry, from all different sectors, critical national infrastructure, nuclear, I mean, everything. can imagine. And everyone wants to use AI for cybersecurity, because of course, we’re just human. But once we have the developers, that was a really interesting point over here about the developer bearing liability. But once the model starts modeling, then it’s gone from the developer. It’s gone from their hands. It’s not in their control anymore. So there was a conversation. And again, from another security institute, the Cognitive Security Institute, really interesting discussion there. And we are human. So a parental relationship, 80% of people we can train, but the other 20%, you know, it doesn’t matter how smart they are, or what, you know, whether they’re on the board, but these are the people that will always click on the link. We know that because that’s human psychology. So do we implement some security and say, okay, we’re going to just implement the security to stop that happening. So let’s secure the system and take out the 20%. I don’t mean that, you know, let’s secure the system so that that can’t happen. And that’s one of those trade-offs that Meledina was speaking about earlier. So maybe the company says, yeah, we’ll have zero trust, we’ll have best practice. But in the end, let’s put some baseline security in just to take away some of that baseline risk. Maybe that’s how we deal with this high risk. And, you know, to get back to our issue of innovation earlier on, it’s a really difficult space, but we can see this unimaginable innovation out there in the future. And it’s just really trying to navigate this difficult space at the moment, so that we can reap, hopefully get to the benefits. Thank you.
Gladys Yiadom: Thank you, Alison. So we’ll take another question from the audience. There’s one lady and then we’ll introduce her.
Christelle Onana: Good morning. My name is Christelle Onana. I work for EODNEPAD, which is the developing agency of the African Union. So my question goes to maybe Meledina and Alison. We discussed earlier, and you say that harmonization happen ideally. So then my question goes of, should we, so last July, the African Union adopted a continental AI strategy. There is quite a lot that needs done on the continent. The countries have different labels of policies and regulation defined. So if there is a continental strategy that has been adopted, it should be implemented sooner or later nationally. Should we then not talk about harmonization because we talk about a system that is global and is difficult to, to may put it to geo-localize to, you know what I mean? That’s one. And what will be your recommendation about then implementing the strategy that has been defined, going about it nationally, engaging with the countries for the development agency that we represent? Thank you.
Melodena Stephens: So you have a mic perhaps, yes. Okay, so I’ll start. I was very pleased to see the strategy, 55 countries, massive, massive, massive. I think we underestimate Africa as a continent and there is a chance now to be actually in the forefront. Now, there are a couple of things that are important to realize between the US private sector model, which is on market capitalization and the European Union model. There are two different things that Africa will have to decide. Are we in it for just the profits for the economy, this thing, or is it also about lifestyle? Because if you look at EU, I remember one of the discussions that was happening in Germany was, Why don’t you list on the stock market? Why don’t you want to be a trillion dollar company? And one of the founders actually said, well, I’m happy with the amount of money that I’ve earned. I can take care of the families. Why do I need to grow? It provides enough. And that’s very different from the other mindsets. That’s one thing that Africa would have to figure out because you’ve got a lot of societal values. Family is important. Society is important. What do you want to focus on? The second thing that I think is important is just to understand what are the assets within Africa. So we know that Cobalt, for example, DRC is a major provider. If we could go across the 55 countries and find unique assets that you could tie in, I think there is a win-win situation for all 55 countries that’s there. This is really important in the future because we see across the world, a lot of countries have assets, but they are sold as commodity products, not value added. And again, I like the EU model because you look at the trade, intra-trade within the EU model, it’s 60 to 70%, which I think is huge. So there is enough for everyone in Africa to benefit if you’re focusing on intra-trade. Non-harmonization, what would come? I think that’s important as standards for interoperability, right? So all of us with USBC, thank you European Union for that. But I think interoperability will be key on how you would want to make it work and even deciding who would be your key markets because who you would sell to will also decide whether you want to align your standards with them. And I think that’s things that you would have to decide at a strategic level.
Dr. Alison: Thanks for that, Melodina. I think I have to come back from an education piece and talk about the ideal world would be something like mobilizing the youth. And there’s all of the IGF youth ambassadors here from different countries. One of a young guy I’ve worked with from Ghana, IGF youth movement there and this vitality. and young people, and really think even going younger and younger, going into schools and doing an education piece that makes sense. So your parents’ business, what happens to your parents’ business, really at that level, so it’s really, it’s understandable the risks that are involved, so that people can embrace the risks and young people particularly can mobilise and get involved and take the actions that they need to, that will help families and help businesses locally. So maybe from the education piece, I mean maybe Kaspersky has something to say on an education piece.
Yuliya Shlychkova: I was just listening to you. Education is indeed important and I think education helps harmonisation. It’s like when people are connected with their minds, it automatically motivates more harmonisation. And I believe that education efforts should also be shared responsibilities that not only governments, but also private sector, university, parents, so that it’s also like a common goal and as a private company ready to contribute.
Gladys Yiadom: Thank you Julia, Melodina and Alison for your comments. Perhaps, Johan, do we have another question online?
Jochen Michels: Currently, we do not have questions online. There is a little bit of discussion between the attendees, but no direct questions to speakers.
Gladys Yiadom: Thank you Johan. We have a question on the audience. Can you ask you, sorry to come by ask your question. So please share your name, organisation and who you address your question to please.
AUDIENCE: Hi, I’m Odas. I’m from… Digital Uganda. We’re based in Kigali, Rwanda. And I want to ask Yulia regarding what you mentioned around data poisoning and open source datasets. So my question is around, have you seen some of these instances where there’s data poisoning and open source datasets and are there tools in the preparatory open source that can be used in security audits of such open source datasets?
Yuliya Shlychkova: We did see data poisoning, unfortunately. Because I’m not a technical expert, so I think I would not be able to move further. But even at the hugging phase, there were some backdoors and so ready to exchange business cards with you and connect with our experts who can provide more information. In terms of AI audit, we also see that this is raising trends. And in Europe, already more companies who are providing audits, adding AI audits in their portfolio. And I was able to chat with some of them. And what they’re saying is that they’re also developing methodology. Their first clients, it’s also their like pilots, pilots are grounded. They’re testing this methodology. So I believe we will see more and more of this.
Gladys Yiadom: Thank you, Yulia. We have another question from the audience.
Francis Sitati: Thank you very much. My name is Francis Sitati from Communications Authority of Kenya, which is a regulator for the ICT sector. My question is about the ethical considerations of AI. When you talk about innovation in AI, you can’t miss to talk about the ethical issues, especially with regard to the psychological effects of developing the data models. We’ve seen big tech companies using proxies, to, you know, leverage the affordable labor or cheaper labor within developing countries. So what do you think are some of the considerations in terms of AI practices, to promote AI practices with respect to the ethical use of AI?
Melodena Stephens: So this is a tough one, right? Because when I look at ethics, I think ethics are great. The line between good and bad is a difficult one. So on one hand I go, I want to increase the level of income. So I come and I choose cheap labor, but I’m also willing to close when I find another cheaper labor source. And this is the challenge we have to face, right? Or I want to introduce AI, but I don’t have any implications on the consequences to environment as an example, water consumption, electricity, e-waste recycling. E-waste is far more toxic than carbon dioxide, but we don’t have enough e-waste recycling centers. So with ethics, I think we need to, and there are many standards. I think the UNESCO has put up one recently at UNGA. They all agreed on certain standards. The problem is again, operationalizing it. So there are guidelines. And I think it’s for us to figure out what does that mean for our country and our people? And I always like it to be people-centric. So if I’m saying transparency, why do I want transparency for my people? And it could be because I want a cultural, I want it to be culturally sensitive. If I think in my culture, a child or someone to the year of 16 or 18, not necessarily 12, then I want it also to be aligned for my culture. Family is important. And I, maybe in my culture, it’s. collective family, it’s uncles, aunts, extended family. So I think translation is the difficulty which we don’t have alignment worldwide. So we have all of these things, we don’t know how to operationalize it, and we don’t know how to go and implement it. So right now at this point, because AI is being perceived as the in thing and because of national security issues, there’s a huge investment in AI. I wanted to mention this, the current tech debt is around 40 to 50%. That means if you put in 1 million into a project, you need to keep half a million for upgrading the system, retraining the system for cybersecurity. We are not considering that and that is leading to a lot of failure. Currently, right now, the AI failure rates is around 50 to 80%. So I just want to share this data set with you. 1.5 million apps on Google and Apple has not been updated for two years. 1.5 million apps. That’s a data vulnerability point. That’s a cybersecurity issue. And in 2022, Apple removed something like a half a million apps. So we’re seeing that we’re starting businesses using AI and the first question is why? What is the benefit for the human being? And the second thing is we’ve not considered we can’t sustain the business. So it becomes a cybersecurity issue. So yes, I think AI ethics, I mean, I’m happy to sit with you separately. IEEE also has a policy on a couple of these things, but they’re all guidelines. We aren’t able to implement it because there are cultural nuances and interpretation.
Gladys Yiadom: Thank you very much, Melodina, for highlighting this. Perhaps Sergio, Julia, any comments? Oh, Alison. No, please. Sergio, please go ahead first and then Alison.
Sergio Mayo Macias: No, no problem. I totally agree that ethics is a grey field. It is difficult to mandate ethics. Let’s say, for instance, if you are hiring people, you are a recruiter, or you are using AI for helping in your recruitment, is it fair, for instance, if you want, let’s say, a German native speaker to develop a system promoting CVs received from Germany? Are you avoiding to use CVs received from other countries? Are you going to read everything in the CV for filtering before calling people to interview? So they are difficult questions. So it is ethic or it is not ethic to define, to develop this type of algorithmics. I was mentioning before the algorithmic fairness. This is something that we have to have in mind, of course, fairness, but fairness is different than ethics. So we should think before developing an AI system if we want to use it for a personal use or for including other people being involved in the use of the AI system.
Gladys Yiadom: Thank you, Sergio. Alison, please.
Dr. Alison: Yes, thanks. This is probably outside of my domain, but I think we discussed earlier that ethics is probably something that’s a cultural norm. So I think maybe ethics for you are slightly different for ethics for different people from around the world. So maybe it’s something you’ve probably already done all of this and thought of this, but maybe something bottom up. What does ethics mean to you? Where does it come from? What are the norms of ethics? And this is probably an education piece at local schools, schools getting involved in consultations and helping you develop those. I’m sure you’ve probably done all of this. and then leveraging, I think, as Melodina was saying earlier, your unique assets, your unique resources with those tech companies, because the tech companies, we know who they are, some of them, well, they’re not, actually, I don’t see any of the exhibition stands, actually, but it’s quite interesting, they’ve got so much weight in the world, but I think if you can look at your assets and say, well, these are our unique assets, and maybe leverage that in this really imbalanced world with those tech companies, maybe, I don’t know, I hope that helps. Thank you.
Gladys Yiadom: Thank you, Alison. Yulia, perhaps, as Kaspersky developed last year, the ethical principles.
Yuliya Shlychkova: Yes, we believe that ethics is important, transparency is important, and also, in addition to mandatory regulation, self-imposed standards also is vital in the whole ecosystem, and we, as a company, developed our own principles, ethical principles, we mandatory declared to adhere to, and I think this is a good practice, and more and more companies, they joined in different pledges, showing their principles, so this has already happened, this is good, but I also wanted to comment that we even internally had this discussion, whether the usage of AI can influence the workforce, because right now, in Kaspersky, we have, like, 5,000 engineers, and our top, top-notch researchers, and we’re really proud of our research teams, because they’re able to discover very advanced cyber-Spanish campaigns, and our researchers are part of the community, which are, like, 100, 300 in the world, so it’s very unique talents, but they all started being regular virus analysts, investigating very simple viruses before they grew up to that level, so we were thinking whether introducing AI to do more simple tasks will kill this journey, maturity journey and actually we ended up with positive thinking because we believe with more AI being used to automate skills like the professional will shift from doing things manually from maybe being more operator of AI model so skills will be a little bit different but still the journey will be there and humans will be required. So at least internally we hope that it will affect human employment but still will introduce more opportunities and different job profiles.
Gladys Yiadom: Absolutely, I think this also has been one of the key questions that we’ve hear in international forum is about the future of work in the context of AI. Thank you very much for sharing that, Julia. We can also take one or two other question. Are there any question from the onsite audience? I don’t see any. Johan, do we have one or two last question? Oh, see, we have one, sorry.
AUDIENCE: Hello, can you hear me?
Gladys Yiadom: Yes, we can.
AUDIENCE: Okay, my name is Paula. I am from GIZ African Union. I think in the presentation, you showed that there was some cyber incidents that had happened based off of AI. But do we have any case studies on cybersecurity incidents based off of AI that have destabilized the nation? For instance, any sort of use of autonomous weapons to attack a particular nation and that.
Jochen Michels: We cannot hear.
Yuliya Shlychkova: But we also started to see more advanced use, used by advanced actors But it can happen in a very persistent manner For example, there is a collection of malware samples for all cyber security companies to refer to And we see that for some time, a malicious actor was sending samples With specific logic So that all cyber security engines later, trained on these samples Would recognize or not recognize this thing I’m trying to explain this in simple words But definitely we see that more advanced attackers are trying also to use these And let’s say to affect machine learning algorithms which are working in cyber security software So that later when they release their highly capable cyber-espionage campaigns The defense technologists would not see it or would act something So unfortunately we will see this more, but this is a race and we’re used to this in cyber security Attackers come in with new technology, we come in with new defense And in defense we also, in layers responsible for anomaly detection We also use a very highly efficient AI which can detect anomalies So we are good, we are on par, so there is hope
Gladys Yiadom: Thank you very much Julia, I think this leads us to the end of the session I would like to first thank our speakers for joining us today Online moderators and participants online and on-site We are available to continue this conversation Please do not hesitate to reach out to us and we’ll be happy to follow up with that The guidelines will be available online, so please also do not hesitate hesitate to check them. Thank you very much.
Dr. Allison Wylde
Speech speed
170 words per minute
Speech length
1832 words
Speech time
645 seconds
Trust in AI is subjective and culturally dependent
Explanation
Allison Wylde argues that trust in AI is not a universal concept but varies based on individual perceptions and cultural backgrounds. This subjectivity makes it challenging to measure or quantify trust in AI systems.
Evidence
Allison Wylde mentions that trust is naturally given by humans, such as children trusting their parents without thinking.
Major Discussion Point
Trust and AI Adoption
Zero trust approaches should be integrated into AI development
Explanation
Allison Wylde suggests implementing zero trust principles throughout the AI ecosystem. This approach requires continuous verification of identities and permissions before granting access or trust.
Evidence
Allison Wylde mentions the complex, dynamic ecosystem of AI with multiple moving parts that need continuous monitoring.
Major Discussion Point
AI Security Challenges
Agreed with
Yuliya Shlychkova
Agreed on
AI security challenges
Gladys Yiadom
Speech speed
0 words per minute
Speech length
0 words
Speech time
1 seconds
Over 50% of infrastructure companies have implemented AI despite trust concerns
Explanation
Gladys Yiadom presents data showing widespread adoption of AI in infrastructure companies. This suggests that organizations are implementing AI technologies despite ongoing concerns about trust and security.
Evidence
Kaspersky study revealing that more than 50% of infrastructure companies have implemented AI and IoT in their infrastructure.
Major Discussion Point
Trust and AI Adoption
Yuliya Shlychkova
Speech speed
123 words per minute
Speech length
2812 words
Speech time
1363 seconds
AI is still software and not 100% safe, leading to cybersecurity concerns
Explanation
Yuliya Shlychkova emphasizes that AI systems are fundamentally software and thus inherently vulnerable to security risks. This leads to ongoing cybersecurity concerns as AI adoption increases.
Evidence
Yuliya mentions registered cases of AI being used by cybercriminals and AI systems being attacked.
Major Discussion Point
AI Security Challenges
Agreed with
Allison Wylde
Agreed on
AI security challenges
AI models can be vulnerable to data poisoning and adversarial attacks
Explanation
Yuliya Shlychkova highlights specific vulnerabilities in AI models, including data poisoning and adversarial attacks. These vulnerabilities can compromise the integrity and performance of AI systems.
Evidence
Examples of attacks include data poisoning of open source datasets, backdoors, and prompt injection targeting AI algorithms.
Major Discussion Point
AI Security Challenges
Agreed with
Allison Wylde
Agreed on
AI security challenges
Open source AI models may introduce new security vulnerabilities
Explanation
Yuliya Shlychkova discusses the potential security risks associated with open source AI models. While beneficial for innovation, these models can also introduce vulnerabilities if not properly audited and secured.
Evidence
Mention of backdoors and vulnerabilities found in open source datasets used to train models.
Major Discussion Point
AI Security Challenges
Education efforts can help build trust and harmonization in AI adoption
Explanation
Yuliya Shlychkova emphasizes the importance of education in fostering trust and harmonization in AI adoption. She suggests that shared educational efforts can lead to better understanding and alignment in AI implementation.
Evidence
Yuliya mentions that education helps harmonization by connecting people’s minds and motivating more alignment.
Major Discussion Point
Trust and AI Adoption
Agreed with
Melodena Stephens
Agreed on
Importance of AI education and literacy
Continuous training on AI risks and best practices is necessary for organizations
Explanation
Yuliya Shlychkova stresses the need for ongoing training within organizations on AI risks and best practices. This continuous education helps maintain awareness and preparedness for evolving AI-related challenges.
Evidence
Yuliya mentions the importance of regular updates to training courses and conducting field exercises.
Major Discussion Point
AI Education and Literacy
Agreed with
Melodena Stephens
Agreed on
Importance of AI education and literacy
Sergio Mayo Macias
Speech speed
0 words per minute
Speech length
0 words
Speech time
1 seconds
The EU AI Act provides a model for regional AI governance
Explanation
Sergio Mayo Macias discusses the EU AI Act as an example of regional AI governance. He suggests that this model could be adapted or considered by other regions developing their own AI regulations.
Evidence
Sergio mentions that some Latin American countries are consulting on the EU’s data spaces model for potential implementation.
Major Discussion Point
AI Regulation and Governance
Differed with
Melodena Stephens
Differed on
Approach to AI regulation and governance
Algorithmic fairness is crucial but challenging to define and implement
Explanation
Sergio Mayo Macias highlights the importance of algorithmic fairness in AI systems. However, he notes that defining and implementing fairness in algorithms is complex and can vary based on context and use case.
Evidence
Sergio provides an example of AI use in recruitment, questioning whether filtering CVs based on language proficiency is fair or ethical.
Major Discussion Point
Ethical Considerations in AI
Melodena Stephens
Speech speed
0 words per minute
Speech length
0 words
Speech time
1 seconds
Lack of algorithmic transparency makes it difficult to audit AI systems
Explanation
Melodena Stephens points out that the lack of transparency in AI algorithms poses challenges for auditing these systems. This opacity can make it difficult to identify and address potential biases or errors in AI decision-making.
Evidence
Melodena mentions the example of Google’s Willow, which performs calculations in minutes that would take supercomputers septillions of years, making it practically impossible for humans to trace or audit.
Major Discussion Point
AI Security Challenges
Africa has an opportunity to develop its own AI strategy and standards
Explanation
Melodena Stephens discusses the potential for Africa to take a leading role in AI development by creating its own strategy and standards. She suggests that Africa can leverage its unique assets and cultural values in shaping its approach to AI.
Evidence
Melodena mentions the recent adoption of a continental AI strategy by the African Union, covering 55 countries.
Major Discussion Point
AI Regulation and Governance
Differed with
Sergio Mayo Macias
Differed on
Approach to AI regulation and governance
AI ethics guidelines exist but are difficult to operationalize
Explanation
Melodena Stephens acknowledges the existence of AI ethics guidelines but points out the challenges in implementing them practically. She highlights the difficulty in translating broad ethical principles into concrete actions and decisions in AI development and use.
Evidence
Melodena mentions various ethical standards, including those from UNESCO, but notes the problem of operationalizing these guidelines in different cultural contexts.
Major Discussion Point
Ethical Considerations in AI
AI literacy should distinguish between digital skills and AI-specific knowledge
Explanation
Melodena Stephens emphasizes the need to differentiate between general digital literacy and AI-specific literacy. She argues that understanding AI requires a more specialized set of knowledge and skills beyond basic digital competence.
Evidence
Melodena points out that current digital literacy often focuses on digital skills training, which is not equivalent to AI literacy.
Major Discussion Point
AI Education and Literacy
Agreed with
Yuliya Shlychkova
Agreed on
Importance of AI education and literacy
Unknown speaker
Speech speed
0 words per minute
Speech length
0 words
Speech time
1 seconds
There is a need to increase AI literacy among professionals and the general public
Explanation
This argument emphasizes the importance of improving AI literacy across society. It suggests that both professionals and the general public need a better understanding of AI technologies and their implications.
Major Discussion Point
AI Education and Literacy
Youth mobilization and education are key to responsible AI adoption
Explanation
This argument highlights the role of young people in shaping the future of AI adoption. It suggests that educating and engaging youth is crucial for ensuring responsible and ethical use of AI technologies.
Major Discussion Point
AI Education and Literacy
Self-imposed ethical standards by companies are important alongside regulation
Explanation
This argument emphasizes the value of companies developing their own ethical standards for AI use. It suggests that these self-imposed guidelines can complement formal regulations in promoting responsible AI practices.
Major Discussion Point
AI Regulation and Governance
AI’s impact on the workforce requires careful consideration of human-AI collaboration
Explanation
This argument addresses the potential effects of AI on employment and work processes. It suggests that organizations need to thoughtfully plan for how humans and AI systems can work together effectively.
Major Discussion Point
Ethical Considerations in AI
Agreements
Agreement Points
AI security challenges
Allison Wylde
Yuliya Shlychkova
Zero trust approaches should be integrated into AI development
AI is still software and not 100% safe, leading to cybersecurity concerns
AI models can be vulnerable to data poisoning and adversarial attacks
Both speakers emphasize the need for robust security measures in AI development and implementation, highlighting various vulnerabilities and the importance of continuous verification.
Importance of AI education and literacy
Yuliya Shlychkova
Melodena Stephens
Education efforts can help build trust and harmonization in AI adoption
Continuous training on AI risks and best practices is necessary for organizations
AI literacy should distinguish between digital skills and AI-specific knowledge
The speakers agree on the critical role of education in fostering responsible AI adoption, emphasizing the need for specialized AI literacy and continuous training.
Similar Viewpoints
Both speakers highlight the complexity of implementing ethical guidelines and fairness in AI systems, acknowledging the challenges in translating broad principles into practical applications.
Sergio Mayo Macias
Melodena Stephens
Algorithmic fairness is crucial but challenging to define and implement
AI ethics guidelines exist but are difficult to operationalize
Unexpected Consensus
Regional approach to AI governance
Sergio Mayo Macias
Melodena Stephens
The EU AI Act provides a model for regional AI governance
Africa has an opportunity to develop its own AI strategy and standards
Despite representing different regions, both speakers advocate for regional approaches to AI governance, suggesting that tailored strategies can be more effective than global one-size-fits-all solutions.
Overall Assessment
Summary
The main areas of agreement include the need for robust AI security measures, the importance of AI-specific education and literacy, and the challenges in implementing ethical guidelines and fairness in AI systems.
Consensus level
Moderate consensus exists among the speakers on key issues, particularly regarding security challenges and the importance of education. This level of agreement suggests a shared recognition of critical areas that need addressing in AI development and implementation, which could potentially guide future policy and industry practices.
Differences
Different Viewpoints
Approach to AI regulation and governance
Melodena Stephens
Sergio Mayo Macias
Africa has an opportunity to develop its own AI strategy and standards
The EU AI Act provides a model for regional AI governance
While Melodena Stephens emphasizes the potential for Africa to develop its own unique AI strategy, Sergio Mayo Macias highlights the EU AI Act as a model for regional governance. This suggests different approaches to AI regulation in different regions.
Unexpected Differences
Focus of AI literacy
Melodena Stephens
Unknown speaker
AI literacy should distinguish between digital skills and AI-specific knowledge
There is a need to increase AI literacy among professionals and the general public
While both speakers agree on the importance of AI literacy, Melodena Stephens unexpectedly emphasizes the need to differentiate between general digital skills and AI-specific knowledge, which adds a layer of complexity to the discussion on AI education.
Overall Assessment
summary
The main areas of disagreement revolve around approaches to AI regulation, methods of building trust in AI, implementation of ethical guidelines, and the focus of AI literacy efforts.
difference_level
The level of disagreement among the speakers is moderate. While there are differing perspectives on specific approaches and implementations, there is a general consensus on the importance of addressing AI security, ethics, and education. These differences highlight the complexity of global AI governance and the need for flexible, context-specific solutions.
Partial Agreements
Partial Agreements
Both speakers agree on the importance of trust in AI adoption, but they propose different approaches. Allison Wylde emphasizes the subjective nature of trust, while Yuliya Shlychkova suggests education as a means to build trust and harmonization.
Allison Wylde
Yuliya Shlychkova
Trust in AI is subjective and culturally dependent
Education efforts can help build trust and harmonization in AI adoption
Both speakers recognize the importance of ethical guidelines for AI, but they differ in their approach. Melodena Stephens highlights the challenges in operationalizing existing guidelines, while Yuliya Shlychkova emphasizes the role of self-imposed company standards.
Melodena Stephens
Yuliya Shlychkova
AI ethics guidelines exist but are difficult to operationalize
Self-imposed ethical standards by companies are important alongside regulation
Similar Viewpoints
Both speakers highlight the complexity of implementing ethical guidelines and fairness in AI systems, acknowledging the challenges in translating broad principles into practical applications.
Sergio Mayo Macias
Melodena Stephens
Algorithmic fairness is crucial but challenging to define and implement
AI ethics guidelines exist but are difficult to operationalize
Takeaways
Key Takeaways
Trust in AI is subjective and culturally dependent, making it challenging to establish universal standards
AI systems face significant cybersecurity challenges, including data poisoning and adversarial attacks
Harmonizing AI regulations globally is difficult due to cultural and regional differences
Ethical considerations in AI development and deployment are crucial but challenging to operationalize
Increasing AI literacy among professionals and the general public is essential for responsible AI adoption
Resolutions and Action Items
Kaspersky has developed guidelines for AI security that organizations can use to improve their AI systems’ security
Companies should consider developing and adhering to self-imposed ethical standards for AI use
Unresolved Issues
How to effectively harmonize AI regulations across different jurisdictions and cultures
How to operationalize AI ethics guidelines in practical implementations
How to balance innovation with security concerns in AI development
The long-term impact of AI on the workforce and job markets
How to ensure algorithmic fairness and transparency in AI systems
Suggested Compromises
Adopting a risk-based approach to AI regulation, similar to the EU AI Act, to balance innovation and security
Focusing on interoperability standards rather than full harmonization of AI regulations
Leveraging unique regional assets and cultural values in AI development strategies
Implementing multi-layered protection in AI systems, combining automated AI security with human oversight
Thought Provoking Comments
Trust is subjective. So maybe I trust you. I think I probably do. I don’t really know you too well, but I trust you. I’m a human. And so our human behavior is naturally to trust. Children trust their parents without thinking about it. And I think that’s one of the issues in business. People see a new technology and they want to be with the top technology, with the new technology. And of course they want to use it really without thinking.
speaker
Allison Wylde
reason
This comment challenges the assumption that trust in AI is a simple yes/no question. It introduces the complexity of human psychology and how it relates to trust in technology.
impact
This shifted the discussion from a technical focus to considering human factors and psychology in AI adoption and trust. It led to further exploration of how to define and measure trust in AI contexts.
We almost see in the wild attacks on every component of AI development chain. Therefore, cybersecurity should be addressed. We need to talk about this and help not to stop AI usage but to do it safely and have basis for this trust in for AI use in the organization.
speaker
Yuliya Shlychkova
reason
This comment provides a comprehensive view of the cybersecurity challenges in AI, emphasizing the need for a holistic approach to security.
impact
It broadened the discussion from general trust issues to specific cybersecurity concerns across the AI development chain. This led to more detailed conversations about security measures and best practices.
If you look at how many policies are there for cybersecurity, I think there are more than 100 countries which have policies. While some of them are on security and they’re looking at algorithmic security, we see recently over the last two years maybe more focusing on critical infrastructure. And there’s two things driving it. One is we’re moving away from individual security or corporate security or industry security to national security.
speaker
Melodena Stephens
reason
This comment highlights the evolving nature of AI security policies and their increasing focus on national security, introducing a geopolitical dimension to the discussion.
impact
It shifted the conversation towards considering the broader implications of AI security at a national and international level, leading to discussions about the need for global cooperation and standards.
We need to provide cybersecurity by default. We cannot send the elephant in the room to final users. We have to define safe spaces for using the AI systems and we cannot expect final users to do it.
speaker
Sergio Mayo Macias
reason
This comment challenges the current approach to AI security by emphasizing the need for built-in security measures rather than relying on end-users.
impact
It sparked a discussion about the responsibilities of AI developers and providers in ensuring security, leading to conversations about potential regulatory approaches and industry standards.
Currently, right now, the AI failure rates is around 50 to 80%. So I just want to share this data set with you. 1.5 million apps on Google and Apple has not been updated for two years. 1.5 million apps. That’s a data vulnerability point. That’s a cybersecurity issue.
speaker
Melodena Stephens
reason
This comment provides concrete data on AI failures and vulnerabilities, highlighting the scale of the cybersecurity challenge in AI applications.
impact
It brought a sense of urgency to the discussion and led to more focused conversations about practical steps needed to address these vulnerabilities and improve AI reliability.
Overall Assessment
These key comments shaped the discussion by broadening its scope from initial considerations of trust to encompass complex issues of human psychology, cybersecurity across the AI development chain, national security implications, the need for built-in security measures, and the urgent challenges posed by current AI vulnerabilities. The discussion evolved from theoretical considerations to practical concerns and potential solutions, emphasizing the multifaceted nature of AI security and the need for collaborative, proactive approaches across various stakeholders.
Follow-up Questions
How can we develop a conceptual framework for trust in AI?
speaker
Allison Wylde
explanation
Trust is subjective and can’t be measured with traditional statistical methods. A conceptual framework is needed to define, measure, and implement trust in AI systems.
How can we address the issue of shadow AI use in organizations?
speaker
Yuliya Shlychkova
explanation
Many employees are using AI tools without organizational oversight, potentially exposing confidential information. Understanding the scale of shadow AI use is crucial for security.
How can we ensure algorithmic fairness in AI systems?
speaker
Sergio Mayo Macias
explanation
Even with good data, the human creating the algorithm must ensure fairness. This is a key point in addressing bias and ethical concerns in AI.
How can we balance national security concerns with individual privacy in AI regulations?
speaker
Melodena Stephens
explanation
This trade-off is crucial in developing AI policies and regulations that protect both national interests and individual rights.
How can we address the challenges of AI security given that AI responses can be different each time?
speaker
Audience member
explanation
Traditional security measures may not be effective for AI systems that produce variable outputs, creating new challenges for vulnerability detection and mitigation.
How can we develop and implement AI-specific protection standards for organizations using applied AI systems?
speaker
Gladys Yiadom
explanation
Current standards mostly cover AI foundation models, leaving a gap in protection for organizations implementing applied AI systems based on existing models.
How can we effectively harmonize AI regulations across different jurisdictions, particularly in Africa?
speaker
Christelle Onana
explanation
With the adoption of a continental AI strategy in Africa, there’s a need to understand how to implement it nationally while considering the global nature of AI systems.
What are the ethical considerations in AI development, particularly regarding the use of cheaper labor in developing countries?
speaker
Francis Sitati
explanation
There’s a need to explore ethical AI practices that balance innovation with fair labor practices and cultural sensitivities.
Are there case studies on AI-based cybersecurity incidents that have destabilized nations?
speaker
Paula from GIZ African Union
explanation
Understanding the real-world impact of AI in cyber warfare and national security is crucial for developing appropriate defenses and policies.
Disclaimer: This is not an official session record. DiploAI generates these resources from audiovisual recordings, and they are presented as-is, including potential errors. Due to logistical challenges, such as discrepancies in audio/video or transcripts, names may be misspelled. We strive for accuracy to the best of our ability.
Related event
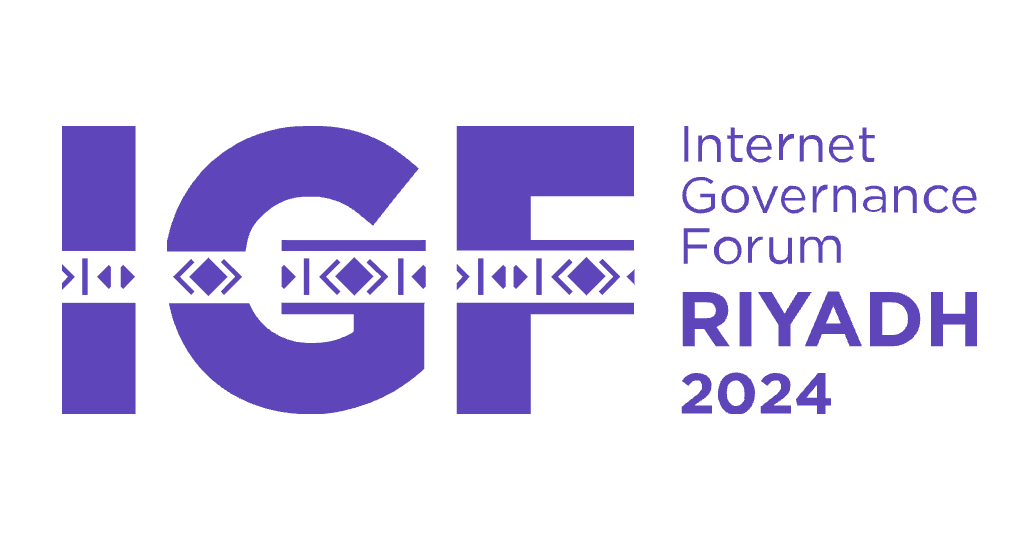
Internet Governance Forum 2024
15 Dec 2024 06:30h - 19 Dec 2024 13:30h
Riyadh, Saudi Arabia and online